This conference call transcript was computer generated and almost certianly contains errors. This transcript is provided for information purposes only.EarningsCall, LLC makes no representation about the accuracy of the aforementioned transcript, and you are cautioned not to place undue reliance on the information provided by the transcript.
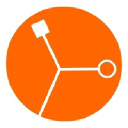
Exscientia Plc
5/26/2022
Hello everyone. My name is Brent and I will be your conference operator today. At this time, I would like to welcome everyone to Accenture's business update call for the first quarter of 2022. At this time, all lines have been placed on mute to prevent any background noise. After the speaker's remarks, there will be a question and answer session. If you would like to ask a question at that time, simply press star followed by the number one on your telephone keypad. If you would like to withdraw your question, again, press star 1. Thank you. At this time, I'd like to introduce Sarah Sherman, Vice President of Investor Relations. Sarah, please begin.
Thank you, Operator. A press release in 6K was issued yesterday after U.S. market closed with our first quarter 2022 financial results and business update. These documents can be found on our website at www.investors.Xentia.ai, along with a presentation for today's webcast. Before we begin, I'd like to remind you that we may make forward-looking statements on our call. These may include statements about our projected growth, revenue, business models, and business performance, including with respect to product development, preclinical and clinical progress, and our precision medicine platform. Actual results may differ materially from those indicated by these statements. Unless required by law, Accenture does not undertake any obligation to update these statements regarding the future or to confirm these statements in relation to actual results. On today's call, I'm joined by Andrew Hopkins, Chief Executive Officer, and Nicholas Krause, VP Precision Medicine. Ben Taylor, CFO and Chief Strategy Officer, and Dave Hallett, Chief Operations Officer, will also be available for the Q&A session. And with that, I will now turn the call over to Andrew.
Thank you, Sarah, and thank you to everyone joining us today. Accenture is off to a strong start in 2022. This is led by several factors. Announcing our strategic collaboration with Sanofi to develop a pipeline of AI design medicines, utilizing the breadth of our end-to-end platform. Our teams have commenced target identification and validation work, and we'll share updates as this progresses. We are also advancing our pipeline with several near-term milestones anticipated. GTA EXS 617, our co-owned program with GTA Pylon, for which we plan to submit a CTA by the end of the year. We also expect top-line data from our Phase 1 Healthy Volunteer Study for EXS 21546 in the first half of this year. What is also critically important to us as a management team, and heightened in today's environment, is that we've maintained a strong balance sheet and cash fund rate. As of March 31st, 2022, our cash balance was approximately $720 million, with an additional $100 million upfront payment from Sanofi received in April 2022. During the first quarter, our operating cash burn was only approximately $10.4 million. This gives us confidence in being able to execute on our near-term business plan with our current cash on hand. Lastly, and the focus of today's call, advancement in building out our proprietary precision medicine platform, including new data generated by the platform that we presented at this year's AACR annual meeting. At our previous quarterly calls, we took you through our balanced business model and our AI drug design technology platform. Today, we are focusing on deep learning-driven precision medicine, and I'm delighted to be joined by Nicholas Call, our Vice President of Precision Medicine. Nicholas will share more about our work on this front and how we integrate in primary tumor sample data throughout our end-to-end system in a way not previously possible for drug discovery and development. As we saw in the groundbreaking EXALT-1 Cleansing File, recently published in the peer-reviewed journal Cancer Discovery, our AI-driven precision medicine platform allows each patient to serve as their own model to understand potential treatment effects. This can yield better treatment recommendations personalized to the individual. In EXALT-1, this technology demonstrated the ultimate impact of significantly better clinical outcomes. In essence, the long-sought goal of personalized medicine. At Accenture, we imagine the impact we can make for patients if we take this approach from the start of discovering a new medicine, integrating patient-led insights throughout each stage to yield more precise, better-informed decision-making and design. This is what we call patient-first AI and has the potential to significantly improve the probability of success of turning science into new medicines for patients. The work we presented at this year's AACR meeting demonstrates tangible ways in which we've been able to bring this to bear. Firstly, with EXX21546, our clinical stage A2A antagonist, we show how a deep learning approach to reveal phenomic endpoints can be integrated with single-cell sequencing. This helps us to move forward towards identifying the patient signatures and potential biomarkers that will help us understand if a treatment is likely to work best for a specific patient, hence potentially enriching our clinical strategy. For CDK7, we applied to a platform to assess our novel AI design development candidate, GTA EX617, through the application of deep learning-driven image analysis. We detailed the molecule's effects on primary tumor samples from patients at single cell resolution, a far more complex dynamic view than what's possible with patient-derived cell lines that are common in the industry. And by using models built from our proprietary human tissue biobank, we were able to reveal ALK-related pathway inhibition as a novel mechanism of action in a subset of ovarian cancers. This means that with the use of patient-focused models, which have higher translatability and can better capture patient heterogeneity as opposed to traditional move-on models, we were able to uncover clinical relevance at the targeted discovery stage. A common thread throughout this work is the potential to increase our probability of success within drug discovery and development. If you recall, improving probability of success, in addition to accelerating the time of turning new science into new medicines and lowering costs, underlies our goal to shift the curve in the economic life cycle of drug development for the industry. It's clearly a relevant and pressing concept in today's environment. And with that, let me turn to today's topic and how we're progressing on this mission. As you can see here, drug discovery as we know it today is a linear or sequential process. One that starts with identifying a target, progressing through molecular design, and into clinical development, and ends many years later potentially with a drug. And by the time this drug is commercially available, it's been likely been over 10 years or more. This sequential approach is not only inefficient, The flaws in either design or other factors may not be realized until a drug is in late-stage clinical trials. We believe the structure of this process contributes to the low probability of success we see across the industry. Further, we believe that the act of drug creation is best described as a patient-first learning problem. What you see here is how we have taken the conventional, sequential way of problem solving to think of drug discovery as a learning problem or a learning loop where all aspects are fundamentally connected. You may recognize this from our prior presentations where we talk about our full end-to-end process. Last quarter, we touched on AI driven molecular design, highlighting our tech for generative design and active learning. Today, we are just focusing on a key aspect of this with our precision medicine platform. Today, the biopharma industry uses mostly cell lines and organoids that have limited translatability into the clinic. And we believe this is why the industry faces a near 96% failure rate. Our goal is to increase the probability of success of new drug candidates by using the closest representation of an actual patient to help inform every stage of drug discovery and development. What's different about what we do is that we actually take the live patient tissues, which we believe has a higher clinical relevance, given it is as close to the actual patient as you can get. Our aim is then to assess from the live patient tissue which molecule would likely work for that patient. We can then bring that data into how we think about selecting targets and use a real patient data-driven approach to AI drug discovery. using our algorithms and generating high-quality, relevant data to design the best, balanced molecule. We are taking the insights from the ExcellentOne trial further by placing the patient at the center of our learning loop, where we can then work to enable and form a platform to potentially identify the best drug for a given patient across a range of indications. This is driven by our AI platform, which learns from every new piece of data and covers everything that we do from targeted identification to the potential for identifying the patients whom we believe will most likely respond in a given clinical trial. We take what was done conventionally in the clinic upstream into drug discovery. So many years before drugs historically would be tested in patients, we can measure its potential impact. And to reiterate, we showed a lot of this at the ACR, including how we can identify novel targets, evaluate the modulation of a complex tumor microenvironment, and work to better understand patient enrichment through biomarkers. I'll now turn the call over to Nicholas, who will walk us through how we have developed this first-of-a-kind technology, validate it in the clinic, and how we are using this tech to inform drug discovery for projects going forward. Thank you, Andrew, and thank you everyone for joining us today. It's my pleasure to walk you through our unique patient tumor tissue-based precision medicine platform and show you how we use it to drive patient-first drug discovery and development. I'd also like to detail the evidence that our concept of bringing the patient into preclinical research may actually support the development of more efficient, better designed medicines. The terms precision medicine and personalized medicine have been around for a while. we talk about precision medicine as giving the right drug to the right patient at the right time. Traditionally, this is based on dividing patient populations into ever smaller subgroups based on biomarkers who are then all given the same treatment. Importantly, this is a concept that is usually not well addressed until drugs reach the clinic. By contrast, here we show our approach to precision medicine based on deep understanding of the treatment effects in an individual patient-by-patient level. Further, we use the knowledge of how patients would like to respond already at the preclinical drug discovery and development stage. This is possible because we can measure drug response directly in live human tumor tissues. So what evidence do we have that drug response measured in live human cancer tissues contains clinically relevant information? As Andrew mentioned, we conducted a study called EXALT-1, where our aim was to determine if we could prioritize effective therapies by testing the activity of drugs in patients' very own tumor tissues. The study was a prospective, single-arm, open-label basket study of 76 patients with a variety of aggressive hematological cancers, and it's the first-ever prospective clinical study demonstrating the clinical impact of AI-driven functional precision medicine. On the left, you can see the setup of our functional precision medicine platform. As you can see, we take primary tissues from patients such as blood, bone marrow, and lymph nodes and expose these tumor tissues to over 100 different drugs. We then use our AI-enabled high-content single-cell image analysis to assess drug effects on these samples. This process is fast with a turnaround of less than five days. For this study, drugs were ranked by their ability to selectively kill cancer cells and not to kill off-target cells. These data were then shared with physicians who chose individualized therapies for these patients. Here on this slide is one of the first patients they exhaled one trial, an elderly late-stage diffuse large B-cell lymphoma patient with no standard of care available. So what you can see here is a sample of the patient's tumor that was taken and exposed to a library of over 100 drugs to determine which had the most selective activity against his specific cancer cells. In the chart, you can see the tested drugs ranked in order of the selective anti-cancer activity. Then, integrating our ranked list and the overall state of the patient, the tumor board selected the second drug from the top. The patient was treated and able to go into complete remission. Most strikingly, he stayed in complete remission for two years. Now, when we look at the full study, the results are also clear. Patients had a significantly longer progression-free survival, or PSS, compared to their prior line of therapy, when taking the individualized therapy prioritized by a functional precision medicine platform. By contrast, when patients were treated with drugs that were not prioritized by the platform, there was no improvement in PFS compared to the prior line. Interestingly, pretreatment performance influenced outcomes, and patients with an ECOG score less than or equal to 1 were more likely to experience improvement in PFS, showing the potential for greater benefits to be realized if this platform was to be used earlier in the treatment paradigm. What this shows is that drugs found to be active in primary tumor tissues have a high chance of being effective in the clinic. We believe this supports the use of primary tumor tissues as highly patient-relevant model systems in preclinical research. This means that before drugs are even tested on patients, we can use our precision medicine platform in drug discovery with the aim of identifying drug candidates that have a higher chance of being effective in the clinic and potentially increasing the probability of success in terms of finding the right drug for the right patients. As for these encouraging results, we intend to expand our clinical research efforts into solid tumors and are planning to conduct an observational study to better understand the potential of our platform in prioritizing effective therapies outside hematology. As we'll talk about later in the presentation, we've already been using solid tumor samples for drug discovery efforts. It's important to note that there's some precedence for using this platform in solid tumors, as there has been some exploratory work from Professor Beren Snyder at ETH Zurich. A small observational study of 14 patients with glioblastoma showed statistically significantly longer PFS and overall survival, or OS, for patients whose tumor tissues were temozolomide-sensitive as compared to insensitive ones. In our observational study, we plan to take patient tissues and send these to our labs for analysis. Separately, the physician chooses a therapy, what we here refer to as physician's choice, without using the assay. The same drug is then tested for activity in the patient's live tissues, and we compare the response in the clinic to the ex vivo drug response. Our hypothesis is that drug response measured in patient's tumor tissues will correlate with clinical outcome. We look forward to updating you on our progress for this exciting study. I'll now spend some time focusing on what makes our patient-served AI screening technology truly unique. in how we screen for drug effects in a highly scalable way in primary tumor tissues using microscopy and quantifying certain cell outcomes such as cell death and cell morphology using the eye-powered image analysis. Approaches used in the past and by many of our peers today have focused on measuring global cell death in bulk tissues. Tumor tissues, however, rarely comprise only of cancer cells. They contain a plethora of immune and between a toxic drug that may kill non-cancer cells, one that is effective and selectively kills only cancer cells. Relying on measurement of bulk tissue death may in fact prioritize molecules that are not effective against cancer cells at all. As a consequence, these approaches often are not translatable in a clinic or do not provide patient benefit. What's needed is a drug that is balanced, must be potent but also selective. In other words, it must have a good therapeutic window. Developing balanced drugs, as you may remember from previous presentations, is an important focus of Accenture through all aspects of discovery, design, and development. To be able to distinguish between broadly toxic and selectively active anti-cancer drugs requires single-cell resolution, which is what we do in our platform. It is this single-cell resolution that has allowed us to be clinically translatable, deliver patient benefit, and differentiation in the industry. So how do we achieve this? As I alluded to, tumors are inherently complex. They're made up of various tumor cells and immune cells that grow in 3D. Our automated microscopy tools allow us to analyze drug responses in these complex specimens with unprecedented speed and single-cell resolution, which is key, as outlined in the previous slide. Importantly, we can do this without fully dissociating the tissue into individual cells, thus maintaining vital parts of the tumor microenvironment and cell viability. In brief, we take tissues, minimally process them to make them imageable, expose them to drugs for no more than four days, which avoids culture adaptation and clonal selection bias, fix and stain the fluorescently labeled dyes that allow us to visualize effects of interest, for example, selective killing of cancer cells. We then take high-resolution images, which generate many gigabytes worth of data per patient, and then process them using computational image analysis. While computational image analysis has existed for decades, our use of AI and our proprietary tech stack makes it possible to process the complex images that we are generating accurately and at scale across many different tumor indications. Here you can see an example 3D microscopic image of a solid tumor sample as an animated stack. It comprises many cell types growing very closely together, which makes it difficult to analyze using conventional image analysis technology. To give just a few examples, to overcome this analytical challenge, we have built highly specialized deep learning models to find nuclei, cytoplasm, measure staining intensity, classify life versus death, cancer versus immune cells. With these models, we can analyze microscopic imaging data faster, more reproducibly and accurately than humans could ever do. This is yet another example where AI helps us to work better and faster at Xentia. Our proprietary platform is specialized with tools highly optimized for a given task or problem, allowing us to not only provide more accurate outputs compared to what general-purpose third-party software systems are able to do, but also to be faster and work with more precision. The aforementioned readouts allow us to count and characterize cells based on well-established features, such as cell surface marker expression. This is sufficient for many translational questions. However, for other applications, we need to and can extract much more information from our images. In our data sets, we see cells of many different morphologies, sizes, and shapes. We believe differences in cell appearance encode important information about drug response going beyond traditional cell death or activation readouts. That morphological changes in the cell actually relate to functional changes. In order to quantify cell appearance, we've developed deep learning networks that generate a mathematical representation of what a cell looks like. We speak of embedding the visual appearance in a feature vector. These feature vectors have many more than two dimensions, but for simplicity, we can map them to 2D space. Every dot on the map is a cell of unique appearance. The closer they are together, the more similar they look. Using this feature or appearance map, we can, for example, quantify how cells change in appearance as we treat them with drugs. On the right-hand side, you can see a plot of how different a cell looks like at increasing concentrations of a drug compared with no drug treatment. We believe these advanced technical capabilities will be essential when looking at drugs that elicit more subtle phenotypes than just killing cancer cells. Importantly, we're developing patient-derived model systems across different tumor tissues and tumor indications, including blood and bone marrow for leukemia, lymph nodes for lymphoma, and malignant pleural effusions of ascites, as well as solid samples for solid tumors. These patient-centric models are used across the drug discovery pipeline at Accenture. As I've outlined, we can use these models to measure therapeutic effect not only on tumor cells directly, but also on immune cells. So not just cell death, but also the impact on the tumor microenvironment. Going back to the loop Andrew described, I'd now like to highlight a few examples of how we use the platform across our value chain to put the patient at the center of all application, ranging from targeted elucidation to biomarker discovery. So here we have an illustration of how we can use small molecule compounds or drugs as tools to identify biology of disease relevance. We use knowledge about that target spectrum and the fact that small molecules typically have multiple targets to our advantage. So, for example, suppose we have observed that molecule A hits targets 1 and 2, molecule B hits targets 2 and 3, and molecule C hits targets 3 and 4. Further, we see that molecule A and B, but not C, have an effect on the patient tissues. What does this show us? Based on this observation, we can assume that target two would be relevant to explore further. This is just a concept. If we go to the next slide, we show you how we applied this with actual data. Of course, this is more complicated now, but the same basic principle applies. On the left is a panel of over eight drugs assayed in seven-point concentrations and triplicates. The heat map shows how strongly and selectively these different drugs kill cancer cells in primary tissue samples of 20 patients diagnosed with ovarian cancer. The darker the color, the greater the drug effect. Looking at the data in more detail, we found a cluster of tyrosine kinase inhibitors that were active across a diverse range of patients. What we then observed is that these molecules shared some conserved targets, ALK and IGF-1R, as well as some downstream ones. This led to the hypothesis that this pathway into space may be therapeutically interesting. Well, the process of evaluating this hypothesis was complementary omics approaches. We can also use our platform for selecting candidate molecules and indications. On the left, we show what it looks like if a certain molecule works in a certain disease and has an effect. Cancer cells are selected to be depleted over immune cells. This would be a molecule to consider studying further in a tested indication. On the right, there's no effect on cancer and immune cell viability, and no respondents have been depicted for these compounds. So this would not be a molecule to study further in a tested indication. Based on this approach, we can determine which molecules actually have activity in certain indications in the preclinical setting in models that are much closer to the actual patient than cell lines, and which may make sense to develop further in the clinic. We'll now turn the focus to biomarkers and how we believe our platform can help us develop better signatures and biomarkers that are clinically relevant for patients. So here we have an illustration of how we use our ability to measure drug response in primary tissue samples in conjunction with omics analysis to identify biomarkers. At our Precision Medicine Center of Excellence in Vienna, we are currently building out our omics capabilities for this purpose even further. In this example, we can see that patients who respond to a certain drug are enriched for a certain mutation in gene 1. We look at gene 2, there's no such association. Typically, the association between real-world genetics and drug response is only starting to be evaluated in clinical trials. With our approach, we can do this much earlier, ideally going into the clinic already with a hypothesis for patient enrichment biomarker in mind. And this is what it looks like in practice. Here we can see patients treated with our CDK7 inhibitor, GTA-EXS617, are stratified clearly into two response groups, distinguished by dose response curves starting to fall off early, and this plateau effect. As a next step, we'll now work towards correlating these responses to genetics to identify signatures that potentially allow us to enrich patients with higher chances of response in future early clinical studies. In the previous example, we looked at a drug that directly targeted cancer cells. However, working in primary human tumor samples that contain not only tumor but also immune cells, allows us to look at immune modulation as well. This is an important advantage of our approach over conventional approaches using tumor organoids, which do not contain immune cells. This is a key differentiator of our platform. The left-hand side, you can see how we applied this approach to our A2A antagonist, currently in clinical development. When we treat patient samples with an adenosine receptor agonist, we see that a key receptor involved in cellular activity and known association with adenosine sensing, CD71, is downregulated. When treated with our A2A antagonist, we see the reversal of this phenomenon, that CD71 expression goes up again. CD71 here was experimented and selected from a number of receptors based on its robust association with pharmacological modulation of A2A signaling. We are now investigating correlating this functional readout with genetic and transcriptomic data to understand differences between patients and their ability to respond to A2A inhibition. Based on this, we intend to refine existing signatures of adenosine-mediated immune suppression for the selection of patients in our future studies of EXS21546 that have a higher likelihood of responding to A2A inhibition. With that, I'll turn it back to Andrew. Thanks, Niklas. So what does this all mean? We believe our precision medicine patient enrichment capabilities, combined with model-informed drug development and real-time learning, will lead to a more efficient clinical development strategy by selecting the patients who are most likely to respond. When a drug reaches a clinic, we have used actual patient data to inform nearly every stage of target identification, drug discovery, research, and development in an effort to ensure medicines that reach a clinic are potentially more effective. The integration of our AI platform with novel clinical strategies will be led by our new Chief Consultant of Medicine Officer, Mike Krams. Dr. Krams joins us with 25 years' experience in innovative clinical trial design at J&J and Pfizer. With this, our goal is to extend our learning approach to drug discovery into the clinic. Ultimately, by creating a learning loop system made up of breakthrough technologies and science, we aspire to a day when the therapies that work best for each of us are available to all of us. And with that, we now open up a call to questions and answers.
At this time, I would like to remind everyone, in order to ask a question, press star followed by the number one on your telephone keypad. Your first question comes from the line of Chris Shibutani with Goldman Sachs. Your line is open.
Thank you. Good morning. Appreciate the background and the additional update on the inner workings of your precision-based approaches. And congratulations on bringing aboard a chief medical officer. Can you talk to us about how you're thinking about, you know, structuring perhaps more specifically to your lead programs? these early clinical studies in terms of I think you comment in general about having quicker, faster, cheaper, better kinds of responses, more targeted, but maybe specifically for the programs that are on this cusp of entering the clinic, maybe a little bit more information would be helpful about how you're thinking about designing those studies. Thank you.
Chris, wonderful to speak to you again, and thanks for joining today. A great question, actually, and it's key to the heart of what Accenti is building with our end-to-end platform here. It's about how we actually bring in the patient tissue models into every aspect of what we do, from target identification work, using these models as part of our drug design process, particularly selecting our late-stage drug candidates, and then moving that into preclinical studies for biomarker selection and patient stratification. We're doing this sort of IND enabling stage so that we're ready to start our phase ones with actually a patient enrichment strategy right from the beginning. We're hoping to do that actually with the work we're doing on CDK7. Nicholas talked about some of that just now. I'm going to ask Dave Hallett in a few minutes to elaborate a bit more as well. But the key thing about it now is that as our end-to-end platform is coming together is that the programs that we're now driving forward, particularly in precision oncology, are actually now sort of native to that platform. So we do see that a lot of our new oncology programs coming forward, actually. This is the strategy that we're following to bring us forward. The other thing I just want to mention before I open this up to Dave is the use of this precision medicine platform and bringing the patient into target selection and then into patient selection is actually a key part of the work we're doing with Sanofi. It's actually one of the key reasons, I think, why that is such an ambitious collaboration. They are choosing to use the whole platform. But what I want to do now, Chris, is open the floor to David Hallett, our COO, just to give you a bit more color on how his team is really using the patient-based assays in the late-stage target selection and clinical candidate selection processes.
Thank you, Andrew. I think I'll start by the concept that we discussed internally, which is that clinical studies should be confirmatory and not exploratory. What that actually kind of means is that you need to generate kind of politically relevant data, so using our patient tissue platform, kind of years ahead of actually before you even think about going into a clinical study. The way that actually then looks in practice, if you think it was manifest within Nicholas's presentation, and also our recent posters at AACR, we talked about A2A and CDK7. This is about kind of an ex vivo preclinical setting is identifying both relevant and clinically practical kind of either biomarkers, these could be gene signatures, that are derived from testing of our molecules and combinations of molecules on relevant cancer samples so that when we actually go into a clinical study that we can actually prospectively select those patients that are likely to respond to our drug rather than actually doing a clinical study and then retrospectively analyzing who or who didn't respond.
Chris, this is Ben. Just one thing to add on. There certainly will also be the quantitative medicine portion to that. So how are we able to use innovative clinical trial structures, innovative statistical models, both to drive home that patient selection point but also make the trial more efficient? Mike has been doing that for years. He ran a large division at J&J that was doing that for all of J&J's portfolio. So it just brings a wealth of experience in that aspect as well.
Great. Thanks for the thorough answer. I'll get back in the queue.
Your next question is from the line of Peter Lawson with Barclays. Your line is open.
Great. Thank you so much. One clarifying question initially just around the IND for the CDK7 inhibitor. When is that expected? Is that year end along with the CTA filing? Hi, Peter.
Good to speak to you today. We are looking to open a CTA on the CDK7 with an IND later in 2023. And actually the full structure of that and how we're thinking of the early stage plans into humans I'm going to ask Dave, actually, to give you a bit more detail on that.
Thank you, Andrew. And hi, Peter. So, Andrew, you're absolutely correct. For practical reasons, as much as anything else, because we have our current strength in clinical operations in Europe, is that our intention is to open a global multinational trial for CDK7, but we will actually start off with the dose escalation phase in Europe under a CTA, as Andrew points. So that's still on track to actually be executed in the second half of this year. And then simultaneously that we would look to then open an IND in the United States in 23 to actually allow us to access U.S. clinical sites for the cohort expansion. So they're the kind of practical outlines of how we think about running the study, Peter.
And the reason for the IND being in 23 is more of a practical component on your side. It's not because of kind of more stringent requirements from the U.S.?
No, it's purely practical ones. As you're aware, the actual preclinical work that we're doing around toxicity, et cetera, is identical. So the actual packages are the same. It's just really around where our strength and depth is at the moment with our clinical team.
And then on the primary cancer tissue platform, for the ability to kind of select approved drugs, is that something you think about that you would monetize for approved drugs, or this is kind of an internal tool that's key to selecting your own drugs? Your thoughts around that. Sure, and it's a great question.
And the EXALT-1 clinical trial data, we're incredibly excited by. It shows the real potential of this technology. Our primary focus right now is to use this technology to design and develop better drugs. That's why we are expanding a range of indications, as Nicola showed in his trial. That means expanding our links and networks and clinicians as we expand the availability of those tissue samples as we build out our biobanks, looking to see how those clinician networks expand from Central and Eastern Europe. into Asia, and hopefully later soon even announce an expansion into the U.S. as well. So that's a key component to it as well, building out those relationships with the clinicians as well. But right now our focus really is to use this technology to design better drugs and be a pride for not just our own platform, but potentially to partners as well who we work with, such as Sanofi who we mentioned. But we absolutely keep an open mind. As we see this developing and as we think about sort of where potentially these concepts of digital therapies and diagnostics could go, it is certainly something that's on our mind to think about where holistic strategy fits in. But I just want to reassure you right now, our primary focus today is to use the technology to develop better drugs.
Thank you. And just on the phase one aid data for the adenosine inhibitor. What's the plan on presenting that? Is that, would we get a press release or is that going to be preserved for a medical meeting? Just your ideas about venue and how you release the data for the adenosine.
Peter, I'm going to have Ben introduce our thoughts on how we want to communicate the A2A results.
Sure. So, Peter, as you know, that will come out before the end of this current quarter. And I would expect normal top-line readouts during that time period where we'll give some indication along the lines of what we've talked about before, so PKPD and safety. And so we will give you some results on it this quarter. And we may save some of the more detailed readout for a medical meeting to be presented later, as would be normal, but you will get some data. Perfect. Thank you so much. Thanks for taking the question.
Your next question is from the line of Vikram Purohit with Morgan Stanley. Your line is open.
Great. Good morning. Thanks for taking my questions. So I had one on the precision medicine platform, going back to the data you highlighted for EXALT-1. Could you remind us which solid tumor types the platform is currently able to work with and what are some of the capabilities you need to build out to be able to accommodate an even broader set of tumor types?
Thank you very much for this question, Nicholas Crowell here. So the EXALT-1 study was run in hematological cancers, leukemia, but also lymphoma, so solid tumors that have a hematological background. We are currently developing the platform in a range of solid tumor indications, including ovarian cancer, lung cancer. We highlighted this on slide 21 of our presentation. And really we follow a very structured approach here of how we go about this, coming really from assay development, as a first kind of milestone, making sure that we can measure dose-dependent drug responses, then really gathering biological data, understanding whether mechanistically what we're seeing in these primary tissues makes sense all the way to going into clinical testing. And really building on our kind of philosophy as a learning company, the more we do this, the more technology we can reuse. For example, our image analysis capabilities, we're now increasingly seeing that they can really be transferred from one tumor type to the next, the more economy of scale we are going to pick up. and really move this forward. So it's really a question of turning the learning wheel and continuing in our path that we have really first charted out with EXALT-1.
OK. Understood. Thank you. And then a follow-up question to an earlier one on 617. So once that study does start up, both those escalation and expansion portions, could you just give us some color on what what the study could look like, what some of the initial indications of focus could be, and what some of your initial thoughts are about the number of sites to enroll, the number of patients to enroll, et cetera.
I'll give you a high-level view of what the study is going to look like. We're still obviously thrashing through the precise details. I won't be able to give you precise study numbers and patients here. The way to think about this is, first of all, start at the level of the mechanism. So reminding ourselves what CDK7 inhibition does within a cellular context. And so from a mechanistic perspective, this is also where the biomarker work is being done at the moment, is that we'll be looking for signatures, so oncogenic alterations in either the retinoblastoma or the MAP kinase pathway. So that's where the mechanism, that's where we'll be looking. If you then overlay that with, well, okay, how does that then tie into the solid tumor indications, is that the solid tumors that are likely to respond to CDK7 inhibitor and that are aligned with that biomarker strategy are things like lung and breast and ovarian. So what we'll be doing over the course of the second half of the year will provide you with a lot more detail about the study design and also, yeah, the questions you just asked, which is kind of precise patient numbers, study centers, et cetera. But hopefully that gives you a flavor of our direction of travel.
And I think the other important component to layer on to that is there will be a lot of prospective biomarker evaluation from the initiation of the first study. So this will be something that's built into it. We won't be looking at patient populations afterwards and trying to do an ad hoc retrospective analysis. So these are going to be biomarker-informed from the start, and we'll also be using our Vienna platform from the start.
Understood. Very helpful. Thank you.
Your next question is from the line of Michael Riskin with Bank of America. Your line is open. Great.
I got a couple. I'll ask them together, though. First, on the Sanofi collaboration, we realize it's been already communicated. You're going through some target verification. Did you give us any early updates on how that's going, any expectations for the updates and that, and how should we think about catalysts? coming out of that as some of those things move through. And then related to that, my second question would be on PopEx Burn, both as it relates to some of the collaborations, but also for your internal programs. You know, as you've got phase one readouts and CTA submissions coming up, you know, how should we think about the rental spend, given your balance sheet, and, you know, related to that, sort of an update on your expectations for that balance sheet, how it's going to play out over the next couple of years. Thanks. Thanks, Mike. Good to speak to you. Two great questions, actually, and also both very relevant in today's environment. The first one on the Sanofi collaboration, as you absolutely pointed out, we're really excited by this collaboration because it really uses the full end-to-end nature of our platform. And, in fact, we're currently exploiting our centribologists, deep learning knowledge graph technology to really prioritize drug targets from the wealth of information that's out there on a range of oncology and immunological indications for Sanofi. And in fact, then taking that further then into our new target validation platform that we've been building out as well. And so we've already been prioritizing several projects of the 15, which we've signed up for to move forward. And to give you a little bit more color on that, I'm going to ask Dave Hallett actually to take the question further.
Thank you, Andrew. So thank you for the question. And absolutely right. So I'm really excited by this collaboration, but reminding the audience that it was only signed and announced at the start of 22. So we're only a few months into that. But already we've made significant progress in identifying and starting to validate targets to kind of come into that collaboration. So at this point in time, we're not actively designing novel molecules per se. We're working collaboratively with our colleagues at Sanofi to really understand, essentially kind of creating a sandbox, if you like, in terms of doing experimental validation, leveraging, particularly leveraging our capabilities in Vienna to add further credentials, if you like, to those targets. But I look forward to updating everybody on this call over the coming months and years as we transition projects out of the exploratory phase into active design. Thanks, Dave.
And on your question of OpEx, it really is one that's very close to the management's heart, actually. It always has been from the day we actually founded the company to think very carefully about about how we balance the ambitions we have in a company with operating a solid business as well. And I think actually that's why you see such a strong focus on business development inside Accenture as well, as well as new technology development. And to give you more detail on that, actually, I want to open the floor to Ben Taylor, our CFO.
Yeah. Hey, Mike. Always great to be catching up. So a couple of thoughts on the numbers. First of all, I don't want to cast this as Sanofi on its own because, remember, we did enter into partnerships with BMS and EQRx and others last year that are actually going at full bore right now and do have a number of those programs in the design phase right now. So we actually did do a lot of scaling already. And even with that, our cash flow from operations this quarter was negative $10.4 million. That's actually a slight decline over our cash burn from operations last year. But remember, the right way to look at it is over longer periods of time. So looking at cash flows from our partnerships as a total this year, We've had about $116.4 million come in from different partnerships. The Sanofi cash hit the balance sheet in the second quarter rather than the first, so you won't see that in the financials. But we've continued to bring in a lot of cash from those partnerships. That's really balancing off our operational burn. So we'll continue to give guidance that our operating burn will increase year over year. So last year we had about 9 million in that operating burn. We'll continue to see that increase from this year, but we are absolutely trying to be very pragmatic about and sensitive, especially in this environment, on how we spend money. We want to drive forward a business model, but we don't want to do it in a way where we ever feel like we have to make business decisions based on making it to the next financing.
Your next question comes from the line of Christopher Zoff with Goldman Sachs. Your line is open.
Hi, thanks for taking the question this morning. As you talk about innovative and efficient trial designs, one thing that might come to mind is early surrogate endpoints that could give you an early signal of efficacy. When you look through ASCO abstracts this year, you see that there's a lot of interest in circulating tumor DNA. Is that something that you think about looking at in these early trials, or are there other surrogate endpoints that you might be able to maybe leverage your preclinical platforms to identify early signals of efficacy? And then I have one more for Nicholas after that.
Thank you, CJ. Great to speak to you again. You're right. One of the key things that happened this year in the first quarter was actually bringing my crowds on board. We're incredibly excited. someone of Mike's real stature as an innovator in the field. Actually, he ran and developed one of the very first adaptive clinical trials when he was at Pfizer. When he joined us, he was running a 500-person organization at J&J of quantitative science. So to bring that kind of intellectual firepower now to Accenture, Allowed us to really think about how to be as innovative in trial design as we have been in drug design. Moving forward is a really exciting new chapter of how the company is developing. Now, an exciting thing as well about the platform build that we, in Vienna, as the precision medicine platform grows is, as Nicholas hinted in his slides, the range of technologies that we're now developing goes far beyond high-content phenomics. We're already building out our genomics capability. looking at single-cell sequencing, looking at transcriptomics as well. So we certainly will be looking at a wide range of potential genetic and other circulated markers, which then would hopefully allow us to position our drug for the best patients possible. It's far more than just high-content imaging that we're now working on. But to give you a bit of a flavor about how our platform is developing into more of a multi-omics platform, I'm just going to hand this over to Nicholas. Thanks very much for this question, and I also followed these presentations with a lot of interest at ACR. So certainly in modern trial designs, I mean, this is well understood, you need biomarkers both for selecting patients to increase your probability of success, but also to understand as early as possible who will respond and who not. So these are certainly all things that we are exploring. The way how the Vienna platform can really or how we are using it at the moment, As we have shown at the ACR poster, it's, for example, for biomarker discovery for patient selection, where really we are trying to understand in a close-to-patient setting how patient tissues respond to drugs. really in a close-to-patient setting, and then analyze all the genomics of these patient settings, i.e., understanding clinically relevant genomic breadth and kind of setter, correlating these together to really get results. biomarker hypotheses for patient selection that we can then later study in the clinic. So rather than doing this once we are in the clinic, we can actually already go into the clinic with a hypothesis in mind.
Yeah, and one thing that I'd add, CJ, is just whenever you think about CTCs or ctDNA or radiomics or a lot of the alternative or more sophisticated ways of trying to measure response. They are providing really important data and potentially elucidating mechanisms. However, we also need to think about how is the drug going to get approved and how is it going to move through. And so we are a data-driven company. We are focused on making the right clinical decisions as early as possible. And really that goes into, if Mike was here, he would have just said that all of the quantitative medicine that we're doing. But you also have to expect that, you know, these trials will be structured to drive towards a normal regulatory approval. And right now the guidelines for that are pretty clear.
Thanks, Ben. Yeah, that's exactly where I was going next with the question. The clinical platforms seem like they are an excellent way to get a good sense of sort of the very precise, immediate impacts of the drug on the tumor biology. As you are thinking about translating to the clinic, How are you leveraging these platforms to kind of get a sense of either resistance challenges through adaptation or sort of acquired mutations or designing for durability in response? As you said, the approval endpoints are going to be much longer term than you might see with just initial ctDNA decrease. Thanks.
So one of the key ways we think about risk, and it is an important thing about how one responds. One of the interesting things actually with some of the studies Nicholas's team has been undertaking is actually thinking about and looking at actually how we can observe how a patient's response actually changes with therapy. A good example would be, we've been looking at an example of how cisplatin sort of resistance has been occurring in some ovarian patients. And it allows us then to really understand actually that once a drug is given to a patient, it actually then does change the actual tumor, you know. And then actually some of these longitudinal studies then allow us to understand that and see then whether a drug is given later actually has a different effect. And actually just going to open the floor here to Nicholas maybe to tell us a bit more about some of the longitudinal studies we're looking at with the platform as well. I guess what we can do here by having the access to the primary tissue is really simulate some of these approaches that you have described ex vivo. Again, at ACR there was a lot of talk about emerging tumor targets, adaptive tumor response, which I find very exciting. And really what is being done there is to test or to analyze how tumors respond by sequential biopsies across clinical studies. These are things that one can incorporate into clinical studies. As we are already sourcing tissues at many different points in a patient's treatment, we can also do some of these functional analyses ex vivo prior to going into a clinical trial. So I think what you're describing here really very nicely integrates with the way how we think about leveraging primary tissues to our advantage to really understand tumor biology in a patient-relevant setting as early as possible in discovery and development. And at the end of the day, CJ, we consider ourselves a learning organization. So as we see these more innovative ways on how we can use the platform, the platform actually has a wide variety of ways we can exploit it. everything from what Peter was talking about with the possibility of how you could use it to help patients directly, to the possibility that, as Nicholas was talking about, how we can then think about using this, actually, to understand more longitudinal responses as well. And that's why I started to have the benefits of these AI-driven systems, patient-focused systems, really allow us to build, within the company, these rapid-loaded loops. That's what drives, actually, the innovation I think we've seen inside Accenture. The more we do, the more we can learn.
Great. Thank you.
There are no further questions at this time. I will now turn the call back over to Mr. Andrew Hopkins.
Thank you, Brent. I'll make a few final remarks in closing. As a company, we continue to use data-reformed approach to carefully direct our resources towards areas that we believe we have the strongest probability of success, These include continuing to build our differentiated AI-powered precision medicine platform and expanding to solid tumors with high unmet medical need. And we're focusing our pipeline on areas where the platform and our learning systems are powered to make the most difference for patients. This involves leverage of full end-to-end system and strong patient-based model systems in areas such as oncology. Thank you all today for joining us. We look forward to sharing our continued progress with you throughout the year. You may now disconnect.
Ladies and gentlemen, thank you for your participation. This concludes today's conference call.