This conference call transcript was computer generated and almost certianly contains errors. This transcript is provided for information purposes only.EarningsCall, LLC makes no representation about the accuracy of the aforementioned transcript, and you are cautioned not to place undue reliance on the information provided by the transcript.
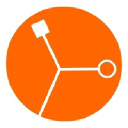
Exscientia Plc
3/21/2024
unmute to prevent any background noise. After the speaker's remarks, there will be a question and answer session. If you would like to ask a question during this time, simply press the star key followed by the number one on your telephone keypad. If you would like to withdraw your question, press star one again. At this time, I would like to introduce Chin Okeka, Associate Director of Strategy and Investor Relations. Chin, you may begin.
Thank you, operator.
Thank you, operator, and welcome everyone to Accenture's full year 2023 financial results and business update conference call. A press release and 20F were issued this morning with our full year 2023 financial results and business update. These documents can be found on our website at investors.accenture.ai, along with the presentation for today's webcast. Before we begin, I'd like to remind you that we may make forward-looking statements on our call. These may include statements about our projected growth, revenue, business models, preclinical and clinical results, and business performance. Actual results may differ materially from those indicated by these statements. Unless required by law, Accenture does not undertake any obligation to update these statements regarding the future or to confirm these statements in relation to actual results. On today's call, I'm joined by Dr. Dave Hallett, Interim Chief Executive Officer and Chief Scientific Officer, and Ben Taylor, Chief Financial Officer and Chief Strategy Officer. Dr. Mike Crams, Chief Medical Officer, will also be available for the Q&A session. And with that, I will now turn the call over to Dave.
Thank you, Chin. In 2023, we continue to make progress in expanding and integrating our technological capabilities. focused our internal pipeline efforts on the highest value oncology targets, and steadily advanced multiple new and existing programs with our major pharma partners. All of these elements position us for success as we work towards our goal of designing and developing better drugs faster. Internally, we are progressing multiple highly differentiated small molecules for oncology, including GTA EXS617 or 617, our CDK7 inhibitor currently in phase 1-2 studies, as well as our LSD1 inhibitor, EXS74539 or 539 in IND enabling studies. With our partnerships, we're progressing multiple programs across an array of disease areas. We recently announced an expansion of our collaboration with Sanofi, to include a new Discovery Stage Accenture-originated program and achieve the first milestone from this partnership. More broadly, and in line with our business development strategy, we inked a new partnership with Merck KGAA Darmstadt in Germany. This partnership, which we signed in September, leverages our precision design capabilities to design and discover novel small molecule drug candidates for challenging oncology and immunology targets. We have also significantly expanded our technological capabilities with the opening of our automation suite near Oxford and the launch of additional functional precision medicine studies. We're currently in the process of fully operationalizing our new automation facility, and once it has reached peak performance, we'll be comprehensively integrating our AI design capabilities with automated experimentation. From this, we expect to achieve material gains in speed, efficiency, and quality across our entire drug design and discovery value chain. The launch of our functional precision medicine studies, XITE-1 and XITE-2, have the potential to support our pipeline with the greater use of human tumor samples in the preclinical development of new drug candidates and translational research. We remain well capitalized with $463 million in cash at the end of the year, providing us with a runway well into 2026 to continue progressing our pipeline and investing in our long-term growth. Through all these updates and developments, the mission of the company remains unchanged. We are focused on improving the lives of patients by creating highly differentiated medicines that solve significant unmet needs. we have repeatedly demonstrated our ability to resolve previously unsolved design problems by efficiently translating scientific concepts into precision-designed therapeutic candidates. This can be seen in our pipeline. In addition to 617 and 539 that I have already mentioned, I'll also highlight EXS4318, a PKC theta inhibitor. 4318 was previously in license by our partner, Bristol-Myers Squibb. and is currently in phase one trials. PKC theta is a high-value immunology target that has been historically challenging for others to deliver small molecules against. We, however, were able to design a highly selective compound with a low predicted human dose and retain significant economics for this program. Our differentiated MALT1 program Precision design with selectivity over UGT1A1 also continues to progress through IMD-enabling studies. And we look forward to providing an update for this program in the first half of this year. In addition to this, we also have multiple discovery programs ongoing. Each of these molecules was designed by leveraging our industry-leading technology platform, shaped with over a decade of experience in the tech-enabled drug design space. We look forward to updating you throughout 2024 about these programs as they mature into the development phases. Our platform was built with our overarching philosophy in mind, that drug discovery is a learning problem, not a screening problem. We use both proprietary as well as public data sets to drive information gain from target ID through to the clinic. Operating at the interface between technology and human ingenuity, we have designed our platform to overcome the key problems seen in drug design. When starting a program, it's important to confirm that the target actually has a strong association with the disease. We use multi-omics data from our patient tissue platform and other sources to find the link between target and disease. Our technology stack then enables our target analysts to properly and efficiently interrogate each target before we proceed to the experimental validation process and drug design. Our pipeline is a physical demonstration of our ability to design well-balanced molecules. Our precision design platform means we have an advantage for targets that have either historically not been tractable or had no design flaws. We're able to achieve this by encoding our desired target product profile at the start of a program and then leveraging multi-parameter optimization to find the appropriate design balance. We have always integrated design with experimental data, and this grounds the models that drive our design to the truth. We now have the capability to automate these experiments with our new automation facility. This will enable accelerated learning, which we will talk about more later. We can further aim for an increased probability of success by selecting the right patient for each treatment option. No two patients are the same, and neither is their disease progression or response to drugs. We use complex heterogeneous primary human tissue samples to get as close as possible to the best model, the human patient. This enables us to pick up signals that would not be possible with current preclinical models. This information is used broadly to support our drug design programs, biomarker identification, and feeds our target identification processes. Operating this way enables us to accelerate the rate at which our systems learn and provides the framework to enable drug design in a much more efficient manner and with a higher probability of success. This increase in speed, probability of success, and cost efficiency have the potential to do what we like to call shifting the curve. If we are able to decrease the cost and time to bring new medicines to patients, we can significantly change the return on investment, or ROI, on each program that we start. By ensuring that these programs result in better quality drug candidates, the demand is likely to increase, further pushing the return on investment higher. This clearly has great economic value. but it also means we can explore more areas from a scientific point of view. Shifting the curve also means more patients will have more choices from the potential expansion of treatment options. This is why I and my colleagues joined Exentea. We are on a mission to make a difference to the pharmaceutical industry at large, and we remain committed to continuing our work here. We are always looking to improve our platform. I will now highlight some of the key updates we've made throughout the year. Internally, we have already started to incorporate large language models, or LLMs for short, into our target identification. One of the applications of our LLMs is the ranking of targets based on their association with disease. Our target ranking LLM includes more than 1 billion parameters and is built from both public and proprietary datasets. The additional benefit of using an LLM is that we can now also rank and then re-rank targets based on additional context that we may be looking for. The example here shows how we have applied this to antiviral targets as part of our pandemic preparedness work. We performed an initial ranking of targets associated with influenza. When performing a general search for influenza, target C is ranked first. Target A is ranked third. and target B is ranked 393rd. However, when we add the additional context of targeting cell binding infusion, these are now re-ranked with target A ranked first and target B ranked seventh. This is a useful insight as cell binding infusion are essential steps in the influenza lifecycle, and disrupting viral entry into the cells can prevent viral replication. Target B has been previously identified as being part of this process and demonstrates that by using more precise prompts, the rankings can be refined towards a specific biological process. These models provide a productivity benefit as they allow us to determine the best targets to prosecute experimentally, as target A currently is. It is important to know that these models do not just have applicability to antivirals, but can also be used in oncology or any other disease indication. This purpose-built model has domain expertise and has outperformed externally available LLMs in our internal benchmarking. Target ranking is a great tool for hypothesis generation. We have also begun to pair this with the large language model enhanced search that integrates a general purpose LLM with our proprietary data sources. This enhanced search allows our teams to drill down into the biological rationale for a target that has been selected. We have focused on the elimination of hallucinations that have been seen with other LLMs and include sources to provide additional background for our target analysts. In this example, we have asked the model, how does CDK7 promote cancer types? The first thing the tool does is identify the keywords or entities in the model. This is important because these words may have synonyms, such as tumor instead of cancer, that we will also want to include in our search. The model then performs a search of the entities and their synonyms and tabulates the results alongside the source material. This generates a first answer to the question and generates follow up verification statements and questions. These statements and questions then go through the search again. This is important because this additional search acts as a form of self interrogation, suppressing hallucinations with additional source material. Once the verification questions have been answered, The model then formulates a final consistent answer. It is important to note that the enhanced search is enabled for conversation. Follow-up questions can be asked based on previous queries, and our teams have already started to use this for the early interrogation of proposed targets. In mid-2023, we announced that we opened our automation facility. Prior to this, we had already started to bring experimentation capabilities in-house. Generating experimental data ourselves ensures its quality, and high-quality, reproducible data is what drives our machine learning models. With 4,500 square feet of studio space, this facility has capabilities in compound management, automated chemical synthesis, automated biological screening, and in time we expect that it will enable us to produce proteins and develop DMPK assays. The use of automation for chemical synthesis and experimentation creates the potential to further reduce cycle times and lower the cost to generate high-quality experimental data. With the fidelity, sensitivity, and reproducibility that comes with automation, we are finding that we are able to develop assays that were not accessible to us just using a manual approach. We believe that we are the first company to have built an automation facility that has the potential to close the loop between AI-led drug design and experimentation. We have integrated software modules that cover AI generative design, active learning, and AI synthetic root design with our hardware. We also believe that we are the first company to develop software that can orchestrate synthesis and experimentation with the computational precision design of compounds. driving the integration of the virtual world with the real world. The experimental hardware is combined with an engineering robotics platform that can physically move samples through the suite. We have developed our own bespoke software to enable this and integrated this with our lab informatics suite. The collection of data is deliberately performed in a format to better enable our machine learning models. These changes also mean behavioral changes in the way our scientists work. Our teams have always been data-led, and this approach further focuses the need for this. With less time required for optimizing and executing experiments, the teams can now be free to spend more of their time on strategic questions and setting the parameters of the experiments being run. The productivity gains from the virtual world are often limited by the reality of the physical world. The logistics and manual work required for the current approach to drug discovery can be a bottleneck. The reason we have made this investment into an automation facility is that while we strongly believe that the use of AI and other computational techniques have incredible value, this alone will not drive productivity through the limitations of the physical world. The maximal impact of AI is achieved when it's deployed in a continuous learning system. This will require the tight integration of AI-driven generative design with high-performance make and test. The speed and quality of our loops is what will determine success. This means integrating learn within the design-make-test loop, and automation has the potential to enable this. We look forward to providing future updates on this platform. In addition to these platform updates, we also want to provide some key updates from our pipeline and how these have the potential to shape the year ahead, starting with CDK7. Due to the dual targeting of cell cycle and transcriptional mechanisms, inhibiting CDK7 could be used for multiple tumor types. The majority of cancers are transcriptionally addicted with semic overexpression, and the impact of cell cycle inhibitors has been demonstrated by CDK4-6 inhibition. The three major CDK4-6 inhibitors generated nearly $9 billion between them in 2022 alone. However, a significant portion of patients ultimately develop resistance. For 617, our CDK7 inhibitor, we are currently in the dose escalation phase of elucidate, an adaptive model-driven phase 1-2 trial. Colorectal, head and neck, pancreatic, non-small cell lung, hormone receptor positive HER2 negative breast carcinoma, and ovarian cancer are all included in elucidate. As you can see on this slide, there are an estimated 75,000 patients diagnosed with these tumor types in the U.S. alone each year. Enrollment for the trial is progressing well, and we expect to move to the dose expansion phase of the trial in the second half of this year. This will be in monotherapy, and we expect to follow this up with combination dose escalation. At the point of transition, we expect to be able to provide data on both the safety and pharmacokinetics of 617. We believe that many failures in the clinic can be predicted based on the suboptimal design of compounds. The table on this slide is color coded to show how close a profile compound is to an optimized target product profile. Green represents no deviation from the product properties ideal range, orange a minor deviation, and red a major deviation. The first two color coded columns are from two CDK7 inhibitors designed by other companies. On the right-hand side, we have 617. You can see that 617 was deliberately designed with a short half-life. This, in combination with its non-covalent binding, enables better control of the duration of inhibition. This is important because extended exposure could lead to systemic toxicity. 617 was also designed with reduced efflux and transporter issues. This is important because issues with transporters will likely contribute to variable absorption and gastrointestinal issues from compound accumulation in the GI tract. This is key because in this table, you can see that both of the compounds on the left appear to have transporter issues. In fact, the Phase I-II compound has reported some safety data with adverse events linked to the GI tract, such as diarrhea, nausea, and vomiting. These adverse events, in turn, may put restrictions on dosing, meaning suboptimal dosing is used. With suboptimal dosing, the drug's full efficacy potential may not be achieved. This stresses the importance of both pharmacokinetic and safety data from our early CDK7 clinical trials. Many of you may remember from our last earnings call the mechanistic rationale for inhibiting LSD1 to treat both AML and small cell lung cancer. At a high level, LSD1 promotes cell differentiation for these cancer types, and in turn either slows the expansion of tumor cells or sensitizes them to cytotoxic agents. We have generated in vivo animal and ex vivo human data that demonstrates efficacy for 539, our highly differentiated LSD1 inhibitor. We now plan on entering into human studies in the second half of 2024 and are undertaking work to identify the optimal patient group to start with. Both small cell lung cancer and AML remain our focus indications for 539. and we are currently performing analysis to find the optimal study sequence. As with all our clinical programs to date, we continue to look to leverage model-informed drug development by incorporating this trial data into our ongoing simulations. This will allow us to assess the benefits and risks of the program to earlier-informed decision-making. We remain excited about our lead pipeline programs 617 and 539. We look forward to providing updates not just on these, but also 565, our MULT1 inhibitor, as well as the other discovery programs in our pipeline. I'll now hand over to Ben Taylor, our CFO and Chief Strategy Officer, to walk us through our partnerships and financials.
Thank you, Dave. In 2023, we signed deals with Merck KGAA and Sanofi that continue to move our partner pipeline forward. In addition to these new deals, we achieved our first discovery milestone with Sanofi, and BMS initiated a phase one clinical trial for our lead program in their partnership, a PKC theta inhibitor called 4318. In total, we have received roughly 230 million from our three major partners, which has been used to expand both our pipeline value and platform capabilities. This number will continue to grow as we hit anticipated milestones over the coming months and years ahead. We intend to be active in business development during 2024. Accentia's fundamental value proposition is efficiently creating drugs that have a greater probability of success than would be possible using traditional methods. That gives us broad flexibility in the types of business development that we can pursue. Our recently announced deal with Sanofi moved one of our internal discovery programs into the Sanofi collaboration with enhanced economics. Even prior to starting clinical development, we have potential payments on that program of 45 million, followed by over 300 million in milestones and high single to mid double digit royalties. We were able to drive this level of economics despite the program's early stage because we were able to show how our technology platform may have solved a major limitation for an existing class of drugs. This is a good example of how an investment into proof of principle can translate into a major economic gain. In the end, our business development is not formulaic. Rather, it is focused on driving the return on investment from the areas where our platform can have the most impact. Now I'll take a minute to close with highlights from our financial results. Full results are detailed in our press release in Form 20F. I'll review the results in U.S. dollars using the December 29, 2023 constant currency exchange rate of 1.27. We ended the year with $463 million in cash, equivalents, and fixed-term deposits. Over the course of 2023, we saved over $60 million versus our original budget. by substantial gains in operational efficiency and prioritizing the programs with the greatest potential opportunity. This has put us in a strong financial position with a cash runway including anticipated milestones well into 2026. This allows us to further advance our differentiated clinical programs, deliver on our partnerships, and expand and integrate our technological capabilities. Our full year net operating cash burn was 150 million. While we are not providing specific guidance, we expect operating cash burn to be less in 2024 than it was in 2023. With that, I will turn the call back to Dave.
Thank you, Ben. Let me summarize 2023 as a year of steady progress, more rigorous pipeline focus, and critically expanded technology capabilities. We expect that our focused internal pipeline may eventually lead to drug candidates of high value and differentiation with a high probability of success that make a great difference to patients, our investors, partners, and also to our employees. Aside from the opening of our new automation facility, enabling the even faster and more efficient internal synthesizing and testing of new AI design molecules, In 2023, we also steadily progressed new and existing programs with our large pharma partners. In 2024, we will continue to advance our internal programs through clinical development. In addition, we look forward to progressing our partner programs to meet agreed deliverables while striking new partnerships and expanding our technological capabilities to cement our leadership in AI-powered drug design, discovery, and development. With that, We'll open up the call for questions and answers.
Thank you. At this time, I would like to remind everyone in order to ask a question, press star, then the number one on your telephone keypad. We'll go to our first question from Alex Stranahan at Bank of America.
Hey, guys. Thanks for taking our questions. Just a couple from us, actually, just on the automation capabilities that have come online. I'm trying to put, you know, maybe a better earmark around the dollar value that we could see this realized in the near term or medium term. You know, I guess, how do you see the facility playing out from a business perspective? Does it have a benefit to COGS or GNA in the immediate impact or is that maybe longer term? And, you know, how about time from discovery to bring new assets to the pipeline? in clinic? And then maybe as a follow-up, how do your current partners view these capabilities? And could this be a good selling point for you to leverage in future partnership conversations?
Thanks. That's a fantastic set of questions. You can probably tell we're collectively really excited about this automation platform and bringing it online. So we opened the facility back in the summer of 23. and started running biological assays during the second half of last year. We're already demonstrating the operational impact. So we expect to see kind of savings in many aspects. So the onshoring of work that we would have typically for the last few years been taking up to contract research organizations. We are bringing chemistry online literally as we speak. And again, we'll expect to see an impact there in terms of, because that's one aspect that we've always outsourced. Biological experimentation has been something we've been kind of growing kind of steadily over the last four years, but chemistry has always been something we've singularly outsourced. We've already started multiple programs on the platform, both internal and with partners. So our partners are already starting to see the benefits of our investment into automation. I think it's important to remember that it's not just cost. That is clearly important. But it's about data quality and data quality for machine learning. And as I think we tried to point out in the slides, it's because we're an organization that fundamentally thinks that drug discovery is a learning problem, is that the speed of learning is really important to us. is that we expect to see a kind of an acceleration, both in the rate at which we learn, that in turn should have a knock-on effect in terms of whilst maintaining quality in design, actually accelerating the speed with which we can bring high-quality candidates forward. I wonder if Ben wants to add any additional color to that.
Yeah, sure. And we're not providing specific guidance on how much of a financial impact it could have, but I'll say – It could be pretty substantial. And we expect the payback period just from a cost perspective alone just to be in a handful of years. This isn't something that needs to run for a decade to pay itself back. So that can give you a sense of some of the cost savings. I'd say the other part to it is on the time savings. This will be in some ways as dramatic of a step as we took with the early AI design work and being able to cut out a massive part of the time requirements for it. So I think what we look at is saying, you know, what can we take down from months to being weeks or short periods of time? And how quickly can we learn to Dave's point? Because If we can get faster learning cycles, we can actually ask more questions and learn more quickly. So you actually do get to a better result, not just that efficiency.
Great. Thanks, guys. Congrats on the progress.
We'll move next to Chris Shibutani at Goldman Sachs.
Hi, good morning. Thank you so much for taking our question. This is Karishma on for Chris. So we were wondering in regards to the Sanofi collaboration, if you had any color or guidance on the cadence of milestones expected in the year ahead. Thank you.
So thank you for that question. So we're very excited about the kind of progress we've been able to make within the Sanofi collaboration. We achieved the first milestone kind of late in 2023. and then added a program that was Accenture originated into that collaboration with enhanced economics around that particular project. So I think that speaks to the kind of progress and the depth we have there. Well, I won't give specific guidance in terms of kind of actual numbers is that we're expecting that the milestones to kind of ramp up over the next kind of 18 to kind of 36 months at a higher kind of cadence than obviously And it's been so far given that the first two years really are about actually preparing the ground, identifying targets, and progressing them on into early development.
Thank you so much.
We'll move next to Peter Lawson at Barclays.
Great. Thanks so much. Thanks for the update. Just a couple of questions around data disclosures. uh your cdk7 kind of when would when should we expect to see clinical data and um and for the dose escalation side of things where we what what should we be focused on and then i've got yes question around five three nine yeah it's mike speaking uh so the um program is currently in its monotherapy dose escalation phase it's a continuous reassessment method
and we'll initially establish a maximal toroidal dose, at which point we will morph into a monotherapy dose expansion. It is at that time point that we will particularly look at efficacy data. That will be an evolving picture. Within this year, we will look but not expect conclusive readouts on efficacy for CEK75.
Will we get to see the efficacy data in the second half?
So I believe that we will have the accumulating data being assessed, but that there will be not sufficient data to make a concrete statement about efficacy in 24.
Is there a plan to kind of present data?
Yeah, Peter, I'll just jump in on there really quick. So you definitely will get PKPD safety data, as you would expect from a phase one trial. And there may be efficacy signals that we could talk about. But if you think about the time period, it wouldn't have been at an effective dose long enough to have durable responses that we'd really want to be able to talk about for efficacy anyway. So I wouldn't expect that. durable efficacy data in the second half of this year, especially because of how dose escalation works. But I do want to emphasize as well, remember that the goal on CDK7 is really a design goal here. This is a mechanism where we've seen CDK4-6 work really well. CDK7 could potentially build off of that, but it's managing that therapeutic window And so that was where a lot of our design work and our platform differentiation come from. So you will actually get to see that out of the phase one data because you'd be able to see that in the PKPD safety data.
Perfect. Thank you so much. It's really helpful. And then on 539, your LSD1 inhibitor, where are you for the IND CTA? Okay.
So we're currently preparing an IND for a first patient study. We're interested in EML, but also small cell lung cancer, and finalizing the cadence of exploration of these indications in conjunction with key opinion leaders, but also in discussions with health authorities.
Gotcha. When do you think... IND or CTA would be filed there?
We are aiming for early third quarter 2024 and are also aiming to have first patient dose before the end of this year. Thank you so much. Really appreciate it.
Our next question comes from Vikram Pirohit at Morgan Stanley.
Hi, good morning. Thanks for taking our question. So we had two One, following up on 539, your release mentions that the XITE2 study that you initiated earlier this year could be used to help progress that program. I was wondering if you could just speak a bit more about specifically how XITE2 could be woven into the development for that molecule. And then secondly, on your appetite for partnerships broadly, what do you think a partnership could look like, either for biopharma or for the tech space in the coming years? What do you think would be interesting for Excientia to form a partnership around in either or both of those spaces? Thank you.
So I will start working on the question regarding the EXCITE studies, whereas EXALT-1 initially just looked at hematological tumors and and we have now excite one also looking into solid tumors and excite two looking at hematological tumors and we are building out our ability to look at data from the tumor samples to see to what degree in these observational studies they can predict outcome And we'll use this in later stages of the program to further establish which combination therapies to explore. Okay, maybe I can... We're getting the partnership question.
Yeah, I can take that one.
Okay.
We absolutely have appetite for more partnerships, and I think you'll see us continue to be very active on the BD. I think one of the things that's been really helpful for us is as our pipeline has advanced and we've started to see some of our own internal programs come up. it actually gives us more flexibility on what we do with some of those programs, like what you saw with Sanofi towards the end of last year, where we took it to a point where we could validate that we were doing differentiated work and we could get enhanced value for that. And so we went in for a partnership of that. It wasn't the same type of partnership that we had done historically, where it was literally starting with a target and then progressing on. It was us taking it forward a little further And the economics on that are terrific as a result of it. So I think there's part of it where we're looking at our pipeline, we're looking at our capabilities and saying, you know, where can we enhance even beyond the value that we've had historically and build off of that? So I think that's a core focus for us. And you brought up the tech side. It's actually interesting. We've got great dialogue with a lot of the big tech names. We're doing sort of the high end of what they'd like to see the entire industry doing. And so there's a lot of great collaboration around that. But it's also interesting because we're not a big data screening company, right? And there's absolutely nothing wrong with a big data screening company. It's just something very different from what we do. We ask very precise questions and we generate proprietary data that is informed to solve those very precise questions. And so our actual compute needs, while they are high end, they are not as big as a big data company would need. And so it's a different type of partnership than a lot of people are looking for. We are not in search of a question. We actually know what the questions are that we go into a project with, and we are on a learning curve to how to solve that. And so we use a lot of different types of AI. We use a lot of different types of technology, and oftentimes they are sort of the cutting edge of where things are going. So there's a lot of ties to the tech community, but it's not maybe the same sort of thing that you've seen out there with other companies.
Understood. Thank you.
And there are no further questions at this time. I would like to turn the conference over to Dave for closing remarks.
Thank you, Operator. And thank you to everyone on the call today for your continued support of Exientia. We remain committed to our mission to fundamentally change how the world designs and develop drugs. The design platform we have been using and evolving for over a decade now the investments we have been making into automation, and the fantastic group of people we have brought together positions us well to achieve this. We are all really excited about 2024 and the milestones ahead of us. Operator, you may now disconnect.