This conference call transcript was computer generated and almost certianly contains errors. This transcript is provided for information purposes only.EarningsCall, LLC makes no representation about the accuracy of the aforementioned transcript, and you are cautioned not to place undue reliance on the information provided by the transcript.
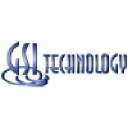
GSI Technology, Inc.
5/16/2023
Greetings and thank you for standing by. Your conference will begin momentarily. We thank you for your patience and ask that you please remain on the line. Thank you. Greetings and thank you for standing by. Welcome to the GSI Technologies fourth quarter and fiscal 2023 results conference call. At this time, all participants are in a listen-only mode. Later, we will conduct a question and answer session. At that time, we will provide instructions for those interested in entering the queue for the Q&A. Before we begin today's call, the company has requested that I read the following Safe Harbor statement. The matters discussed in this conference call may include forward-looking statements regarding future events and the future performance of GSI technology that involve risks and uncertainties that could cause actual results to differ materially from those anticipated. These risks and uncertainties are described in the company's Form 10-K, filed with the Securities and Exchange Commission. Additionally, I have been asked to advise that this conference call is being recorded today, May 16th, 2023, at the request of GSI Technology. Hosting the call today is Li-Lin Xu, the company's chairman, president, and chief executive officer. With him are Douglas Shirley, chief financial officer, and Didier Lasserre, vice president of sales. I would now like to turn the conference over to Mr. Xu. Please go ahead, sir.
Good day, everyone, and welcome to our physical fourth quarter and full year 2023 financial results earning call. The 2023 fiscal year was filled with many positive developments, new partnerships, and progress toward achieving our goals. We also experienced setbacks and unforeseen delays on several fronts with APU, We learned a lot during the year about the stressful market GeminiOne can reasonably pursue with our team, given our limited resource. However, we recently have made significant strides in leveraging third-party resources to help identify users, resellers, and OEMs. These resources are proven valuable in helping us identify opportunities for capturing revenue and increasing awareness of the APU's tremendous capabilities. We have also sharpened our focus for Gemini 1 to leverage our resources and prioritize near-term opportunities, such as synthetic aperture radar, OSAR, and satellites where we have a superior solution. We understand these markets and know whom we can support and help with our offering. Another focus application for Gemini One is vector search engines, where our APU plugin has demonstrated enhanced performance. To this end, we have dedicated more resources and prioritized the target customers that have expressed interest in leveraging our solution. Our data science team has been busy working on the SaaS search project with one leading provider, and we plan to pivot to other players in the space once we have met our deliverables with the first owner. Looking ahead on our roadmap, we will build upon the work we are doing today in future APU versions to address large language models. or LLM, for natural language processing. Vector search engines are a fundamental part of ChatGPT architecture and essentially function as the memory for ChatGPT. Large language models use deep neural levels, such as transformers, to learn billions or trillions of words and produce text. This is another reason that vector search appropriate focus application with the APU. Additionally, we are improving our search and AI SaaS platform to support our go-to-market strategy for search. We intend to use this tool to develop more potential partnerships, like open AI plugin integration that we recently launched, and with other open source decentralized search engines. that use machine learning algorithms and vector search engines. The increasing size and complexity of enterprise datasets and the proliferation of AI in all aspects of business are driving rapid growth in these search engines. Encouraged by the positive reception of our APU plug-in by several key players, we are optimistic about generating modest revenue from this market in the physical year 2024. For both of the Gemini OneFocus applications I have just mentioned, SAR and Fast Factor Search, we have set specific revenue goals that we aim to achieve this physical year. Our L-Python compiler stack has brought progress in the past quarter. Our L-Python compiler stack is designed to offer Python's development advantage by delivering C's high performance without compromise either. Although our current focus application do not require a compiler, we have a beta version in use currently and are on track to release a production-ready version later this year. Your Python will demystify the APU for any Python OC developer. I'm excited to launch this. We are on track to complete the table for Gemini 2 by this summer and evaluate the first silicon chip by the end of calendar year 2023. We aim to bring this solution to market in the second half of 2024. Gemini 2's design will provide significant performance enhancement with reduced power consumption and latency. This feature will extend the future addressable market for the APU to larger markets, such as edge applications, Fast Factor Search, LLM, and Advanced Driver Assistance System, or ADAS. The last one being the vertical we could go after with a strategic partner rather than directly. Gemini 2 is built with a TSMC 60nm process. The chip contains 6MB of associated memory. connected to 100 megabytes distributed with 45 terabytes per second bandwidth, or 15 times the memory bandwidth of the state-of-the-art parallel processor for AI. This is more than four times the processing power and eight times the memory density compared to Gemini One. The Gemini APU is built with big processing. which allows fully flexible data format application and inherent advantage versus other parallel processors. Gemini 2 is a complete package that includes a DDR4 controller and external interface for PCIe Gen 4 by 16 and PCIe Gen 4 by 4. This integration solution allows Gemini 2 to be used in foldable edge applications while still providing significant processing capabilities. In simple terms, Gemini 2 combines different components together, allowing to be used in less expensive devices, while still being powerful enough to handle demanding tasks at the edge of a network. To another way, Gemini 2 brings data center capability to the edge. This means that computationally intensive applications can be done locally, For example, ADAS, delivery zone, autonomous robot, and the UAV, or unmanned aerial vehicle, and satellites. Another application for Gemini 2 could be IoT edge application, like critical infrastructure, or process requiring a reliable and efficient operation. For example, wind farms to mitigate failure mode that can lead to significant financial losses, or operational disruptions. Gemini 2's combination of high processing power, large viewing memory, with tremendous bandwidth and low cost solution provides the best-in-class solution for AI applications like fast vector search, a growing market driven by the proliferation of big data and the need for fast and accurate processing. Recently, we were growing a new patent for Gemini 2's in-memory food adder, which is a basic building block to allow Gemini 2 to perform high processing power. We are thrilled to announce that we are currently in very early stage discussion with the top cloud service provider to explore how Gemini 2's foundational architecture could deliver performance advantage. Just this year, we have seen the disruptive impact of large language models to understand and generate human-like language, like ChatGVT, Microsoft Bing, and Google's Bar. As the boundary of natural language processing continues to be pushed, we envision abundant opportunity in this market for Gemini 2 and future versions of the APU. We believe that we have merely scratched the surface of the potential of large language models and the transformative impact they can have across numerous fields. Large language models attention memory requires very large built-in memory, a very large memory bandwidth armchair. The state-of-the-art GPU solution has built-in 3D memory to address the high capacity memory requirement, but has a poor memory bandwidth for adequate memory access The limitation is going to get worse as large language models are progressing. Gemini chip architecture has inherently large memory bandwidth. It is natural migration to add 3D memory for the next generation Gemini chip to address large memory requirement. This substantial improvement potentially translates into orders of magnitude better performance. As a result, we could be strongly positioned to compete effectively in the rapidly expanding AI market, standing ahead of the industry-leading competitors. Our resources and teams are focused on applications where we have a high probability of general revenue to capitalize on Gemini 1's capabilities. So we bring Gemini 2 to market. We will be more experienced in approaching target customers and creating new revenue streams. We are formulating our roadmap for the APU, which holds tremendous potential. With the future versions, the APU has the capability to cater to much larger markets, and the potential opportunities are quite promising. In parallel with our board of directors, we are actively exploring various options to create shareholder value and remain fully committed to drive sustainability. growth and innovation in the year ahead. Thank you for your support and for joining us today. We look forward to updating you on our progress in the coming quarters. Now I will hand the call over to Didi, who will discuss our business performance further. Please go ahead, Didi.
Thank you, Lilien. As Lilien stated, we have sharpened our focus on a few near-term APU revenue opportunities. In addition, we have strengthened our team with a top data science contractor whose primary job is to accelerate the development of our plug-in solution for the high-performance search engine platforms that Leline mentioned. We have also begun working with a company that offers custom embedded AI solutions for high-speed computing using Gemini 1 and Gemini 2. Another critical development to improve our market access for the APU has been adding distributors. We are pleased to announce that we have added a new distributor for our radiation hard and tolerant SRAM, but also our hardened APU for the European market. In addition to our partnerships and focus on near-term opportunities, we plan to build a platform to enable us to pursue licensing opportunities. This is in the very early stages, and we have work to do before we formally approach potential strategic partners. That said, we have a few preliminary, I'm sorry, we have had a few preliminary conversations on determining what is required to integrate Gemini technology into another platform. This would allow us to identify the specific performance benefits for partners' applications to ensure effective communication of the problem we solve in their system or solution. We recently demoed the Gemini One for a private company specializing in SAR satellite technology. They provide high-resolution Earth observation imagery to government and commercial customers for disaster response, infrastructure monitoring, and national security applications. The satellites are designed to provide flexible on-demand imaging capabilities that customers can access worldwide. They recently provided the datasets to conduct comparison benchmarks on the Gemini 1, and we are commencing the process of running those benchmarks. SAR is one market we anticipate that we can generate modest revenue with Gemini One this fiscal year. GSI was recently awarded a phase one small business innovation research, also known as SBIR. SBIR is a United States government program that supports small business R&D projects that could be commercialized for specific government needs. For this contract, we will collaborate with the Air and Space Force to address the problem of edge computing in space with Gemini 1. Gemini 1 is already radiation tolerant, making it particularly well suited for space force missions. This contract is a milestone for GSI technology as it will showcase the APU's capabilities for the military and other government agencies and provide great references for similar applications. We have submitted other proposals for a direct to phase two project and other SBIR proposals are in the pipeline. On that note, we received verbal confirmation just this morning that we have been awarded a research and development contract which could be worth up to $1.25 million to integrate GSI's next generation Gemini 2 for air and space force mission applications. This revenue will be recognized as milestones are achieved and a typical timeframe is 18 months to two years. Once this agreement, I'm sorry, once the agreement has been finalized and executed, we will issue a press release with full details. Let me switch now to the customer and product breakdown for the fourth quarter. In the fourth quarter of fiscal 2023, sales to Nokia were $1.2 million or 21.8% of net revenues compared to $2 million or 23.1% of net revenues in the same period a year ago. and 1.3 million or 20% of net revenues in the prior quarter. Military defense sales were 44.2% of fourth quarter shipments compared to 22.3% of shipments in the comparable period a year ago and 26.2% of shipments in the prior quarter. Sigma quad sales were 46.3% of fourth quarter shipments compared to 47.6% in the fourth quarter of fiscal 2022 and 45.2% in the prior quarter. I'd now like to hand the call over to Doug. Go ahead, Doug.
Thank you, DDA. I will start with the fourth quarter results summary, followed by a review of the full year fiscal 2023 results. GSI reported a net loss of $4 million, or 16 cents per diluted share, on net revenues of $5.4 million for the fourth quarter of fiscal 2023, compared to a net loss of $3 million, or $0.12 per diluted share, on net revenues of $8.7 million for the fourth quarter of fiscal 2022, and a net loss of $4.8 million, or $0.20 per diluted share, on net revenues of $6.4 million for the third quarter of fiscal 2023. Gross margin was 55.9% in the fourth quarter of fiscal 2023, compared to 58.6% in the prior year period and 57.5% in the preceding third quarter. The decrease in gross margin in the fourth quarter of 2023 was primarily due to the effect of lower revenue on the fixed costs and our cost of goods sold. Total operating expenses in the fourth quarter of fiscal 2023 were $6.9 million compared to $8.1 million in the fourth quarter of fiscal 2022 and $8.5 million in the prior quarter. Research and development expenses were $5 million compared to $6.5 million in the prior year period and $5.5 million in the prior quarter. Selling general and administrative expenses were $1.9 million in the quarter ended March 31, 2023 compared to $1.5 million in the prior year quarter and $3 million in the previous quarter. Fourth quarter fiscal 2023 operating loss was $3.9 million compared to an operating loss of $2.9 million in the prior year period and an operating loss of $4.8 million in the prior quarter. Fourth quarter fiscal 2023 net loss included interest and other income of $101,000 and a tax provision of $191,000 compared to $47,000 in interest and other expense and a tax provision of $21,000 for the same period a year ago. In the preceding third quarter, net loss included interest and other income of $61,000 and a tax provision of $84,000. Total fourth quarter Pre-tax stock-based compensation expense was $515,000, compared to $714,000 in the comparable quarter a year ago, and $654,000 in the prior quarter. For the fiscal year ended March 31, 2023, the company reported a net loss of $16 million, or $0.65 per diluted share, on net revenues of $29.7 million, compared to a net loss of $16.4 million, or $0.67 per diluted share, on net revenues of $33.4 million in the fiscal year ended March 31, 2022. Gross margin for fiscal 2023 was 59.6% compared to 55.5% in the prior year. The increase in gross margin was primarily due to product mix. Total operating expenses were $33.5 million in fiscal 2023 compared to $34.9 million in fiscal 2022. Research and development expenses were $23.6 million compared to $24.7 million in the prior fiscal year. Selling, general, and administrative expenses were $9.9 million compared to $10.2 million in fiscal 2022. The decline in research and development expenses was primarily due to the cost reduction measures announced by the company in November 2022. The operating loss for fiscal 2023 was $15.8 million compared to an operating loss of $16.4 million in the prior year. The fiscal 2023 net loss included interest and other income of $202,000 and a tax provision of $372,000 compared to $60,000 interest and other expense and a tax benefit of $45,000 a year ago. On March 31, 2023, the company had $30.6 million in cash cash equivalents and short-term investments with no long-term investments. Compared to $44 million in cash, cash equivalents and short-term investments of $3.3 million in long-term investments at March 31, 2022. Working capital was $34.7 million as of March 31, 2023 versus $45.8 million at March 31, 2022 with no debt. Stockholders' equity as of March 31, 2023 was $51.4 million compared to $64.5 million as of the fiscal year ended March 31, 2022. Operator, at this point, we will open the call to Q&A.
Thank you. And if you would like to register for a question, please press the 1 followed by the 4 on your telephone keypad right now. You will hear a three-tone prompt to acknowledge your request. If your question has been answered and you would like to withdraw your registration, please press the one followed by the three. And one moment please for the first question. And the first question comes from the line of Raji Gill with Needham. Please proceed with your question.
Hi, this is Nick Doyle for Roger Gill. Two questions on Gemini 2. Are all the costs related to the tape out and then the test and volume production contemplated in your current outlook? And then could you expand on what kind of applications you're seeing traction in with that Gemini 2, specifically anything in ADAS and then using the large language models? Thanks.
Yeah, in terms of R&D spending, yeah, most of what we're spending today is on Gemini 2. You know, we have the hardware team here in Sunnyvale and the software team in Israel. And there will be a tape out in the first half of fiscal 2024 for Gemini 2. It'll run probably about $2.5 billion. Other than that, the R&D expenses should be similar to what we've seen in the most recent quarter.
And regarding the applications, you cut out. Were you talking Gemini 1 or Gemini 2?
Gemini 2, please.
So Gemini 2. So Gemini 2, ADAS, as we discussed in the conversation before, is something we want to address, but we most likely will use a partner to do that. And as far as the large language models, as we discussed, we certainly feel that the Gemini technology, you know, the advantage in the technology certainly will be applicable there. And so whether it's, you know, start with Gemini 2 or if it's also customized with the Gemini 3 is to be determined.
Okay, that makes sense. And then just a quick one. Did you guys – Did you say if there is a timeline, is there a timeline for the RadHard roadmap for the product you mentioned in the EU?
The RadHard and RadTolerant SRAMs are available today. We have done some testing. Boy, it's been at least a year and a half we did the testing on the APU Gemini 1 specifically. It came back very favorable. But the beam was a little bit off that day, so it was limited the tests we could do. So we are actually going to do the full complement of radiation testing in the second half of this year. So we have all the data requirements for the folks that will be sending it into space. So officially, the APU will be rat tolerant sometime by the end of this year.
Excellent. Thank you.
And the next question comes from the line of Jeff Bernstein with TD Callen. Please proceed with your question.
Hi, guys. Just a couple questions for me. Just wanted to make sure I heard right. You brought on a consultant that's helping target applications for Gemini 1. Is that right?
They're specifically helping us write the interfaces for some of the fast vector search platforms that are out there.
Gotcha. And then you said there's a custom embedded AI solutions supplier, and that guy's going to now integrate Gemini 1 into some high-performance compute solutions for clients. Am I getting that right?
Partially. So it's not limited to Gemini 1. It's Gemini 1 and Gemini 2 combined. And they have a multitude of different potential applications, ranging from SAR to satellite applications to marine search and rescue. There's a lot of different applications that they're looking at it for. Some of the cases they'll be able to use essentially are LIDA boards, but in many cases they will be developing their own ultra-small boards for some of these applications that you know, our boards are considered a little too big for those applications. So it's a multitude of different applications, and it will be for both Gemini 1 and Gemini 2.
Gotcha. Okay. And then as far as the large language model kind of applications, I think there's two potentially, correct me if I'm wrong. One is just to run queries as opposed to do training and just run queries of these large matrices together. quickly and at low power. And I guess the other one has to do with making training more efficient by being able to not redo matrices over and over again as you do new learning. Is that right? And which are we talking about here today? Having some light again.
Yes, our primary target will be to do the search, which is the inference top. Okay, we are not... we are not on the training path. Okay, but if you can do search efficiently, you can help the training. Okay, like we can do zero-shot training, okay, or single-shot training, which means you don't really need to train the data set. You know, if you have a first query coming in, we don't recognize, we can store into our memory chip And the second time, a similar item comes in, then you can recognize it right away. That's very different from traditional training. Traditional training, you have to run the whole model, whole data set over again. That's very, very time-consuming. So if you can do zero-shot training, you have the capability to do that, then you can save the training process tremendously.
That's great. Thank you.
And the next question comes from the line of Oren Hirschman with AIGH Investment Partners. Please proceed with your question.
Hi, how are you? Good. So one of the things that the Gemini architecture, you know, in memory processing architecture is very good at, which really wasn't of tremendous interest when you first introduced Gemini, was this natural language processing thing. And all of a sudden, you know, the whole world has changed and you've got things like cat GPT and other similar types of NLP situations where it's actually exactly fits in to what you do best. Like, I guess, you know, it sounded like from one of the prior comments on the last question that you're actually having code and drivers written to be able to optimize the use of Gemini one and certainly Gemini two. this application because i would think that one of the simple applications where you could sell a lot of boards is just simply on the acceleration where everybody is having difficulty using gpus because they're not this is not where a gpu shines on an ai side in terms of the nlp in order to accelerate something like chat gp gpt uh what's the question okay yeah yeah what yeah what what question the question is, isn't that is in fact, is that a priority in terms of what you're working on to be able to introduce your own acceleration board to do it with partners or, or, you know, is, is in fact, uh, you know, great application. It sounds like certainly so far on the call that it's a great application for the Gemini APU.
Okay. Uh, I think I discussed this on, on, on my statement, uh, The biggest challenge for the large language model are two forms. First one, you need a very large memory. The second one, you need a very high bandwidth memory. Those are two very difficult things to achieve. I think today in the market, nobody has this solution, the good solution. So just as I mentioned, we do have... very exciting discussion with, we call it, a larger cloud service provider, and to see how we can help from our Gemini foundational architecture to see how we can help to move this thing forward. Okay, we already have a very, very good memory bandwidth. Okay, as I mentioned in my statement, I say we are 15 times memory bandwidth of the today's state-of-the-art GPU or parallel processor. And that's our inherent architecture. So if we can add this one to the high memory capacity, then this is really the solution nobody in the market can provide. So now we're very excited. We try to explore this advantage we have and see where we can go from here.
Do you have any ideas when you will have the coding for the interface to be able to demo the type of acceleration gains that we're talking about with something like a chat GPP or something like that, so customers can actually see some type of benchmarking, even with Gemini 1 and maybe a simulation until Gemini 2 is ready? When will that code be ready? I know that's what you mentioned you're working on.
Yes, yes, yes. You know, the OpenAI, they have a plug-in. Okay. So basically, you can put your software to plug into the main machine, and then you can utilize the existing model and then do the plug-in. So right now, we are working on it. Okay. And Gemini 1, definitely, and Gemini 2, you know, follow on. And at least they can... you can extrapolate from how well those are working and then extrapolate to the future.
Any ideas when we might see some benchmarks in the coming months?
I would think maybe a quarter or two, we'll have something to tell you guys.
Okay. And just a related question, but even more futuristic. You know, there's talk of doing something similar to, let's say, and there are a number of projects, and in fact, even you had an early project with MUVI to be able to show off what you can do in terms of visuals as well. And I guess my question is, taking that same natural language processing and doing it on a visual level is beyond belief in terms of computationally intensive, but also well-suited for what you guys do. Is anybody talking about doing anything like that? Obviously, you did that early demo, which impressed a lot of people. But obviously, that's even a step beyond almost what people have dreamed of today. But you can't do that using current architecture. So any thoughts on that from a futuristic perspective? And will that need a Gemini 3? Or can that even be done in a Gemini 2? And then one last follow-up question.
So you're asking whether what we want to do in the future generation?
No, specifically the more important part to me is just in terms of incredible visual search capabilities, almost like NLP search capabilities on visuals. You did that impressive early demo with MUVI, and there have been some other experimental projects, and people all over the world are starting to do experimental projects on massive amounts of visual data. Any more thoughts as to... You know, that's obviously very suitable or uniquely suitable for what you do versus just von Neumann or just GPUs for that matter. Any other interesting projects like that movie project? And I know it's a bit futuristic, but has anybody done more in terms of that massive type of visual search, comparative visual search using NLP for visual search using Gemini?
Yeah, we look at that. With our, just I mentioned, with our... with our department, we extend the architecture advantage of Gemini architecture. We look at the one workload. We can be, if we have enough memory, we will be 10 times faster than any solution exists today, okay? So that's why we are saying, hey, we have this inherent advantage there, but the thing is we don't have enough built-in memory for that. If we can combine for the future roadmap, if we can put enough memory into it, that's what you are looking for, although making performance better than existing solutions.
On that note, a closing question, just in terms of what nanometer geometry is being used for Gemini 1, Gemini 2, and what you're thinking for Gemini 3. And obviously, that will affect what you just discussed in terms of the ability to pack in memory and et cetera. If you can kind of tell us more about that, and then just one follow-up, and that's it for me. Thank you so much.
Yeah. Today, Gemini 1 is 28 nanometer, and Gemini 2 is 16 nanometer. And if we look at the future, today's state-of-the-art GPU is 4nm, right? If we look at the future and then we do 5nm, and then we build in the 3D memory in there, because the only way you can get high-capacity memory with reasonable bandwidth is 3D memory. So if we put in the 3D memory with 5nm, we will be able to make it better.
So this is the follow-up question, which with understanding that in terms of Gemini 3, but knowing that Gemini 2 is going to be the platform coming up here shortly, I mean, the key platform, in terms of your ability to accelerate NLP, again, not visual stuff, forget about that futuristic question, but here today in terms of accelerating NLP applications and chat GPT, et cetera, Is Gemini 2 got enough in it so that you're competitive slash even superior on that type of application to, you know, a leading edge GPU, optimized GPU, like a Hopper style GPU? Have you passed that with Gemini 2? And the question only is, can you leapfrog it even further? That's my last question.
Thank you so much. There are two things, big memory capacity or big memory bandwidth. Okay. We have one of them. So if any workload can fit into our chip, we'll be the best solution out there. There are many, many cases like that. Even the chat GPT, it doesn't have to be a humongous data set. So it can be a smaller data set, and the data set can fit into our chip. We will be number one in the market.
Okay, great. Thank you so much.
And as a reminder to register for a question, press the 1 followed by the 4 on your telephone keypad. The next question is from the line of Luke Boyne, private investor. Please proceed with your question.
Hi. Good to be back. I hope you all are well. It's a very exciting announcement and development. Great to hear all the comprehensive layout there. Just for really kind of minor clarifications and going a little bit broader with the near-term potential, wondering if your Amazon Web Services server offering is capable of fielding, say, like a Just a broader range of companies, of potential end use cases that could more or less play around with your service without having to go through more complex processes of embedding or other integration processes. Just plug and play and see what you can do for their application, especially thinking about vector search, but also rich data, like was mentioned, maybe for metaverse, maybe for dense registration. things like that. Yeah, wondering how you're seeing the potential to expand Amazon Web Services or a similar offering on, say, Azure or other clouds, and especially how that would relate to an earlier rollout of Gemini 2 from your own facility, your own servers on those clouds.
So we've started, as we've discussed in the past, we've started the integration with OpenSearch. And so that's ongoing now. It's really – and we have already set up our own servers for that. We have some here in our Sunnyvale facility, some in our Israeli facility, and then we also have some at an off-site facility that's directly across the street from AWS West, and it's directly connected. So we have that in place with the Gemini 1. Over time, obviously, we would migrate those to Gemini 2. So those are in place. We do have some SAR demos that people can run off of those remotely. It's not set up yet to be able to load your own data. It's the data sets that are already in there which you can run. And so we're not at the point yet where you can enter your own data, at least not large data sets. But that is certainly the direction we're going. We're just not quite there yet.
Do you have a timeline on when you would be able to roll out those interactive features and capacities?
We're shooting for this year. Some of the examples you brought up is going to be we're going to get some help from this data science contractor that we have on board now. So it's something we're trying to roll out second half of this year.
Excellent. All right, that's all I have. Pipeline's loaded. Yeah, appreciate y'all.
Thanks, Luke.
And the next question is a follow-up from the line of Jeff Bernstein with TD Cowan.
Hi, yeah, just wanted to see if you could give us an update on the ELTA SAR application and what's going on there.
Yeah, so as you recall, we did the POC with them, and it was a very broad POC. It could be used for different vehicles or vessels. It could be used at a lot of multitudes of heights from 100 meters to much, much higher, obviously, into space. And so the initial program they were looking at for us was was just a single laptop, I guess you could call it that. And they had already been using a GPU, and so they're using the GPU still for that program. There's a fall-on program that they're looking at as for now, and so we're going through that process with them. So it won't be another POC because we've already done one, but it'll be kind of a bit of a different project than what we were working on with them. but it'll still be under SAR, and it'll still be the same algorithm, so it should be a simple integration.
Okay. And then just wondering about waiting to hopefully get some space provenance on the RadHard SRAM and wondering if you guys have any visibility now on when that launch might happen or is it permanently scrubbed?
No, it's not permanently scrubbed. We follow up. I get your frustration because I'm with you on this one. So it's not scrubbed. There were multiple programs that they, when I say they, there was a few, as we talked, defense contractors were using it. There have been a couple of the programs that have been scrubbed, but the larger ones we're looking at have not been scrubbed. They're certainly still out there. It's just, They've just been pushing out the launch dates, and we're just not getting a good feel for exactly when the next launch is going to be. Originally, we know they were delayed because they couldn't get some critical components, and now it's just a matter of, you know, getting them to actually do it. So the answer is we're still optimistic about it. It's just the timing is elusive for us on when it's actually going to happen.
And so can the European distributor kind of do anything on the rad hard piece, or are they stuck with just doing rad tolerant until you get that space provenance, or is it a different approach in Europe?
Oh, no, they're definitely going to be going after everything. So the folks that we've already sent parts to that we're looking to get heritage, it's really just a heritage part. And the heritage is just a signal to the world that says your parts have been have been launched into space and they work. And so it's really, it's an additional check mark in a box for a lot of these folks. But it doesn't change the fact that our parts are already internally qualified to work up there. So we know they will work based off of the testing that we have done. So this European distributor is going to be finding additional opportunities for us. I mean, the folks that we were looking to do the heritage for the short-term launches, those were U.S.-based companies. We have shipped some rad-tolerant and at least one rad-hard to a European customer, but they were not the ones we anticipated to get us the initial heritage.
Okay. Okay.
All right. Great. And then any update on some of the scientific applications? Has, you know, Weissman Institute come back for more boards or any, you know, analogous type customers in, you know, pharma, medtech, biotech, universities, et cetera?
Universities, yes. So we're candidly not spending a lot of time on that market. The Revenue opportunities for the other markets we've discussed today are larger. We do have two universities that, well, let me think. Yeah, there are two different applications for two different universities. They're looking at them for genomics. And so they will be running, they'll be essentially doing the algorithms and doing the write-up. But personally, we are not spending much effort ourselves. We've already done a plug-in specifically for the Biovia program. And so it just doesn't make sense for us, based off of our limited resources, to spend more time developing more algorithms for more platforms. The revenue volumes there just aren't as great as they are in other markets we're addressing.
Makes sense. Makes sense. Thanks.
There are no further questions at this time. I will now turn the presentation back to the hosts.
Thank you all for joining us. We look forward to speaking with you again when we report our first quarter physical 2024 results. Thank you.
That does conclude today's conference. We thank you for your participation and ask that you please disconnect your lines.