This conference call transcript was computer generated and almost certianly contains errors. This transcript is provided for information purposes only.EarningsCall, LLC makes no representation about the accuracy of the aforementioned transcript, and you are cautioned not to place undue reliance on the information provided by the transcript.
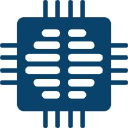
Innodata Inc.
5/7/2024
programs with this customer we announced less than two weeks ago on April 24. We expect that these programs will ramp up over the next two months. While our customer agreements typically contain early termination upon notice provisions, we believe this customer is committed to a significant multi-year LLM strategy from which we stand to benefit. In fact, we are in discussions with this customer regarding potential new programs and expansions beyond what we have announced so far. We also signed two additional big tech companies, a large prominent generative AI company and a large prominent consumer facing ISV investing substantially in generative AI foundation models. As a result of these new wins, we now serve seven big tech customers. We believe we will continue to grow with these customer relationships in 2024 and that we may grow some of them, possibly quite substantially. For big tech customers, we provide a broad range of services to support their generative AI programs. This includes creating instruction data sets, which you can think of as the programming behind large language models. It also includes human preference data used in reinforcement learning and reward modeling to align models to human preferences and build guardrails against toxic biases and harmful responses. In a blog post last month that accompanied a major release, one of our large big tech customers stated that the quality of these instruction data sets has an outsized influence on the performance of their models and that some of their biggest improvements in model quality come from carefully crafted instruction data sets and multiple rounds of quality assurance. This statement crystallizes why we have become the partner of choice for such customers. We believe we are well positioned to anticipate big tech's changing needs and to grow with them. It is evident that big tech's aspirations extend beyond today's predominantly -to-text English language models. We foresee expansion in terms of multimodal models, domain and task-specific models, models natively built in more than 30 different languages, and models capable of complex reasoning. All of these dimensions will require modeling with the kind of data that we create. We believe we are still in the early innings of this journey. I encourage you to read the latest quarterly earnings transcripts from the MAG-7. Generative AI is a prevailing theme with promises of more Gen-AI models, more Gen-AI in products, and commitments to multi-year investment cycles and CAPEX increases to support aggressive AI research and product development. We believe the emerging enterprise market, which we call the middle layer, consisting of companies across verticals that seek to adopt generative AI technologies, to be another important growth factor for innoDATA, one that will ultimately dwarf the big tech market for us. In parallel with executing strategies to penetrate big tech, we are taking steps to prepare for what we foresee as a likely explosion in the enterprise space. We believe we are very well positioned due to our intimate knowledge of the Gen-AI roadmap of the large tech companies, which has enabled us to gain exceptional domain expertise in the future product needs of the enterprise market. We believe that enterprise adoption is about to enter a Cambrian period of explosive growth as a result primarily of three technology developments now underway. I'll explain and illustrate each with examples. Today, enterprise users of Gen-AI are mostly using ChatGPT as a standalone application. We'll call this level zero use case. For example, if I'm an HR director at innoDATA tasked with revising innoDATA's employee handbook, I can prompt ChatGPT to write a first draft of the vacation policy. Companies are now shifting from this level zero to what we think of as level one. We think of level one systems as those based on retrieval augmented generation, or RAG, which we believe are likely to become better performing for reasons I will explain shortly. RAG systems couple search technology and prompt engineering. With such a level one system, an innoDATA employee might prompt an innoDATA HR chatbot with a request like, please summarize for me innoDATA's vacation policy. A search engine working behind the scenes would then retrieve innoDATA's vacation policy from a large document repository and insert it into the prompt as context with an instruction to the LLM to answer the question primarily based on the inserted policy. RAG-based systems are about to become more useful as the latest crop of -to-be released LLMs offer significantly expanded context windows. The context window refers to the amount of retrieved information that can be included with a prompt. By including more context, the chatbot can become more consistent, relevant, and useful. One of the big tech companies is about to release a new model with a context window that is eight times larger than that of OpenAI's GPT-4 turbo, enabling you to include, for example, 3,000 pages of documents in a single prompt. Today's advanced expert augmentation systems are, for the most part, RAG-based systems that combine generative AI with humans in the loop to deliver improved productivity. In a few minutes, I'll give you an example of such a system we started working on for a customer in the quarter. We believe a second technology development called agentic workflows will enable what we'll call Level 2 systems. With an agentic system, rather than asking a question to a chatbot, you present a goal to a virtual agent.
Your
virtual agent then accesses multiple backend systems and LLMs talk to each other to accomplish your goal. Agentic workflows really open up the kinds of things you can ask computers to do with LLMs. With an agentic system, an -a-data employee might ask a virtual -a-data agent, please look up how many days off -a-data employees get, check how many days off I have left and request a week off around my son's graduation so long as there are still available hotels in Boston. Imagine that. Now while the full realization of agentic workflows may be years away, we believe incremental progress is being achieved and will likely accelerate. The third development that we believe will accelerate enterprise adoption is that the cost of training and serving models is likely to go down dramatically, making it possible for enterprises to train and serve models at scale. Once this happens, we believe that companies are likely to want to fine tune their own models rather than relying on rag-based architectures. We'll call these level three systems. Level three systems will support more complex use cases and enable sensitive information to be processed in private clouds or on-premises rather than being served up as context to third-party foundation models. We intend -a-data to offer enterprise all of the services they require to navigate the journey from level zero to level three and beyond. This will include custom development, integration, and fine tuning services as well as managed services, services around data readiness and data governance, and industry-specific workflow platforms. We are not alone in our thinking that the enterprise market for generative AI is about to explode. In a report last year, Bloomberg estimated the market for generative AI-focused IT services will grow to nearly $22 billion by 2027 and to nearly $86 billion by 2032, representing a 100% compounded annual growth rate for the 10-year period from 2022 to 2032. To position ourselves to drive enterprise growth, we are expanding our talent base, creating new accelerators, and winning new reference engagements. This quarter, one of the largest legal information companies in the world engaged us to develop a new LLM-based workflow system for their complex operational processes spanning legal and regulatory law in multiple European countries and multiple languages. This is an example of what I referred to a few minutes ago as an advanced level one system. Our implementation uses GPT and a combination of several techniques, including chain of density, prompt engineering, and a vector database with similarity matching. Our generative AI-enabled workflow system is expected to enable the customer to drive significant operational savings across high-cost processes that previously relied entirely on humans with language and legal expertise. This quarter, we also delivered a generative AI-powered tool that gathers -the-fly insights from large-scale textual data, contextually analyzes the data for specific areas of interest, and performs language translations. The technology aims to increase organizations' efficiency by ensuring knowledge workers are equipped with the intelligence needed to make informed decisions. We built it into our Agility Public Relations platform, where we call it Intelligent Insights. We made intelligent insights generally available to Agility customers in the quarter, and it has been well received. To build the solution, we utilize rag-based prompt engineering. We recently demonstrated it as an accelerator to one of our large banking customers, and it inspired a POC that we are now executing. Agility revenue in the quarter increased .5% -over-year. We have over 1,400 direct customers, and we're generating cash. We've been a leader in the industry rolling out cutting-edge generative AI functionality that is bending the productivity curve for PR and communications professionals. We started early last year with the release of PR Copilot, a generative AI implementation that helps people write press releases and media pitches. In Q1, we announced the general availability of Intelligent Insights. We are planning another five significant generative AI feature releases over the course of the As a result of what we believe is our generative AI leadership in the PR space, in the first quarter, we converted over 35% of demos to wins, up from less than 20% prior to implementation of our generative AI roadmap. Our customers told us that one of their biggest challenges was that they needed more hours in the day. With our generative AI innovations, we're making tactical PR a less labor-intensive process, giving our customers the time back they need for strategic thinking. For both the big tech market as well as the enterprise market, we see additional opportunities in model safety evaluation and responsible and ethical AI. We began working on trust and safety for one of our big tech customers in Q4 2023, providing model assessment and benchmarking services, which help ensure that models meet performance, risk and emerging regulatory requirements. We learned a lot from the work and development we've been doing on this engagement, so we decided to share our learnings, tools and innovation with the market more broadly. Just a couple of weeks ago, we announced our release of an open source LLM evaluation toolkit, together with a repository of 14 semi-synthetic and human-crafted evaluation data sets that enterprises can utilize for evaluating the safety of their large language models in the context of enterprise tasks. Using the toolkit and the data sets, data scientists can automatically test the safety of underlying LLMs across multiple harm categories simultaneously. Developers can understand how their AI systems respond to a variety of prompts and can identify remedial fine-tuning required to align the systems to the desired outcomes. We expect to release a commercial version of the toolkit and more extensive, continually updated benchmarking data sets later this year. In Q1, we won two additional engagements for LLM safety and evaluation, one for a hyperscaler's own foundation models and one for an enterprise customer of the hyperscaler through the white label program we have in place with the hyperscaler. In addition, in Q1 2024, we started pilots for a new customer and an existing customer around LLM trust and safety. I'll conclude with this. We believe we have an incredible opportunity in front of us. We believe we have the talent, capabilities, and scalability to support the world's leading companies' efforts to build AI models and services and to help enterprises advance AI and generative AI technologies. We believe we can drive -in-class growth over the next several years and maintain our early leadership position in generative AI services. Moreover, we believe we can accomplish this without the need to raise equity, to incur debt, or to burn cash. This year, based on our current growth forecast, we intend to invest approximately $3.5 million in recruiting costs to scale our business and approximately another $3 million in new sales, marketing, and product development talent. The recruiting costs relate to the significant increase in revenues we expect this year and will not be incurred next year to support that revenue going forward. The investment in sales, marketing, and product development are incurred to continue our growth We anticipate that they will yield revenue and profitability benefits primarily next year and beyond. We anticipate approximately 70% of the recurring of the recruiting costs to be incurred in Q2 and most of the OPEX investment to be incurred in the second half of the year. We are making these investments while simultaneously driving -over-year growth in adjusted EBITDA and building cash on our balance sheet. At the end of Q1, our cash balances were $19 million, up from $13.8 million at the end of Q4 2023, driven by positive cash flow from operations and tight working capital management. I'll now turn the call over to Mariz to go over the numbers and then we'll open the line for some questions.
Thank you, Jack, and good afternoon, everyone. Let me briefly share with you our 2024 first quarter financial results. Revenue was $26.5 million, up 41% from $18.8 million in the same period last year. Net income was $1 million or $0.03 per basic and diluted share, compared to a net loss of $2.1 million or $0.08 per basic and diluted share in the same period last year. The adjusted EBITDA was $3.8 million, compared to adjusted EBITDA of $0.8 million in the same period last year. Our cash and cash equivalent and short-term investments were $19 million at March 31, 2024, and $13.8 million at December 31, 2023. We currently have an unused line of credit of $10 million with $9.2 million as borrowing base. So thank you, everyone. Paul, we're ready for questions.
Certainly. At this time, we'll be conducting a question and answer session. If you would like to ask a question, please press star 1 on your telephone keypad. A confirmation tone will indicate your line is in the question queue. You may press star 2 if you would like to remove your question from the queue. For participants using speaker equipment, it may be necessary to pick up your handset before pressing the star keys. Once again, please press star 1 on your phone at this time if you wish to ask a question. And please hold while we poll for questions. The first question today is coming from Aria Cole from Cole Capital. Aria, your line is live.
Yes, thank you. Again, congratulations on the good sales results and the good feedback you're getting from customers who are giving you additional business. Jack, one quick question. Clearly companies can grow organically. They can also grow through acquisition. I was trying to get an update on your thought process for how important do you think acquisitions could be as a part of your growth going forward?
Aria, thank you for the question. So the business plan that we're currently executing successfully is an organic only plan. We've got a strategy in place that we think we can successfully deliver through organic growth and through the resources that are available to us and that we're able to augment our team with. From time to time, we will kick the tires on potential inorganic opportunities. But I think the important thing is that we're not dependent upon that for growth. We recognize that those come with risks that are not necessarily risks that one incurs with a, or get primarily organic strategy. And that's the way we're executing right now.
Understood. And then the follow-up is looking at organic growth. When you look at the financial employees, what sort of sales per employee can you generate when you're primarily providing services for these large technology companies? And what is the gross margin range you think you can achieve when people's time is being optimally utilized?
Sure. So we target, at a consolidated level, we target about 40% adjusted gross margin. When you back out the one-time recruiting charges that relate to the revenue that we're scaling up for, and if you back out sales and marketing investments that we plan to have to be returning on that investment in the following year. From a revenue per employee, we don't track that, although it is notable, and it's not lost on us, but our revenue employee has gone, or with the large language model work that we're doing is probably four to even five times in excess of what it's been historically with the business process management services.
Got it. And just finally, in terms of employee retention, you're going to be in a more competitive marketplace for employees in the future, and I'm just wondering what you're putting in place to try and make InnoData a place that people really enjoy working at, where your churn, hopefully our turnover employees, will be kept to a minimal level.
So for the most part, over the years, churn has not presented a problem for us that we weren't able to manage. We were able to keep it at a level that was way below that of most of our competitors. Just this past quarter, we were elected as one of the best places to work in two different locations. So we believe that we will be able to manage it, and we're very excited about the, obviously, very excited about the growth opportunity in front of us.
Okay, great. Well, thank you, and best of luck.
Thank you. Thank you. The next question is coming from Tim Clarkson from Van Clemens. Tim, your line is live.
Hey, Jack. Obviously, a great quarter. Just want to go over some of the basics for some of us Luddites here. So, you know, when I originally realized that InnoData was getting involved in artificial intelligence, the one thing that was really helpful for me in understanding why you guys are successful is just, you know, the accuracy, and the top guy from IBM explained it to me that at IBM, they had a 75% failure rate, largely because of inaccuracies. And what I'm hearing is you guys make like three mistakes and 20,000 annotations. Could you just, you know, talk about how that's really, it seems simple, but it's a real foundational skill. You're doing more than that, obviously, but that really is what initially separates you from the competition in a big way.
Tim, thank you for the question. So absolutely. I think data quality, you know, has been proven to lead to great model performance, and we have customers, as I mentioned a few minutes ago, who with their new releases are attributing their incremental improvements to their data quality, and we're working for those customers. So you know, we're thrilled that, you know, we're producing those great outcomes for our customers and we're seeing that those great outcomes are leading to, you know, expansions and growth. In terms of, you know, LLMs, there are three critical ingredients. There's compute, there's data science, and then there's, you know, data engineering work that we do. Given the investments that these companies are making and the kind of risk that they're taking and the strategic emphasis that they're placing on these developments, it's critical to them to have the kind of data that will result in the models that they, and the accuracy of the models that they're building. So you know, we think we're very well positioned, we're doing great work, and we think the future is very bright.
Right. Now, just as kind of an extension to that idea, I mean, one of the challenges, obviously, in ADATA historically was, you know, you do a project and then it ended and there wasn't a durability of revenues. Can you comment on how durable you think this new business model is going to be into the future?
So I think it's extremely, you know, early days still, you know, we see lots of developments that are taking place. I mentioned several of those. We believe that we're still in the early innings of executing all of these opportunities. We're starting to now talk to our customers about multimodal models, about multiple languages, more complex reasoning tasks that they want the models to be able to take on, domain and task specificity. You know, all of these things are early for them and we think we're early in our partnerships with them such that we're going to be able to drive multi-year growth as a result of their multi-year investments. At the same time, we're lending more companies and we're getting into the enterprise opportunities. So we think there's a tremendous amount of growth opportunity in front of us.
Right. Now, historically, you know, back in the, I don't know if you call it the good old days, but the old days when you guys were peaking on projects, you know, you're doing 20 million. I remember netting 15, 20% after tax and, you know, it seems like you've got these one-time expenses with sales investments, but separating those out, it seems like there's certainly a potential to have 15 to 20% pre-tax margins once you guys get onto a solid run rate.
So, yeah, I think that that's, you know, certainly what we're pursuing. We think the opportunity is there. We also think it's important, you know, as I mentioned, to be investing in our growth. We believe that we have an opportunity in front of us to create, you know, a truly great company. And, you know, we're very excited about that.
Now, these investments would be directly tied into AI then, right, on that part of the business.
Exactly right.
Right. Great. Well, good luck. It's an exciting future. Thank you.
Thank you, Jim. Thank you. The next question will be from Dana Buska from Felton. Dana, your line is live.
Hi, Jack. Hey, Dana. Congratulations on a wonderful quarter. I have just a couple of questions for you. You talked a little bit about the demand for training on enterprise data. Can you talk a little bit more about that? And are you seeing more enterprises look to train their own models?
I think we're going to see more enterprises training their own models when the cost of doing so comes down, which we believe is inevitable. For the most part, what enterprises are looking to do now is to use their own data as context within RAG implementations. And even with that, we see a lot of opportunities that are now being piloted and POCs very early days in terms of getting things into past development and into implementation. We think we're going to be there in order to help accomplish that. Again, quality of data that feeds those models and the kinds of integrations that become possible, especially with large context windows, is going to improve the results of those models and what can be achieved with them. We're very well positioned to help enterprises move things from POCs into development, and we're doing that now today.
Oh, okay. Great. And along those lines, I seem to come across articles about how companies are coming up with tools and software to speed up fine-tuning and doing trust and safety. Could you talk a little bit about how that may affect your business, and do you consider that to be a threat?
No, we consider that to be an opportunity. The more success we have, and the world has it, moving things from POC into development, the more acceleration we will see in the technology and in the opportunities that we have. We are among the companies who are developing capabilities around trust and safety. We're very excited about that. Again, it's a data-driven initiative and a data-driven approach that we're taking that we believe will help companies along in that path.
Okay. Excellent. That sounds exciting. And when you're looking at hiring these new recruiting costs, could you talk a little bit about hiring the new people? Could you talk a little bit about what type of positions you're looking to hire?
So, we're hiring very broadly. We're hiring people who have the ability to help us build the kinds of data sets that we require to train these models. A lot of the people that we're hiring are language experts or they're domain experts. They're linguists. They're people with backgrounds that enable them to appreciate nuance and language. As we think about these models, it's language that is used to program them. And the more fine-tuning we can do around the nuance of those languages, the better performing the models are.
Okay. Great. And then one question about agility. It looked like it grew about 20% last quarter. Is that a growth rate that you are targeting for this year or is that growth rate that you think is sustainable?
So, I think it was about 15%, if I'm not mistaken.
Dana, it was 17%. 70%.
Yeah, so I think 15% to 20% is certainly what we would aspire to. We could do a lot more with more reinvestment in it, and that's something that we would probably be considering at some point. But right now, we're very focused on capital allocation relative to the service opportunities we have with large tech companies.
Okay. Excellent. It sounds very exciting. Thank you for taking my questions.
Thank you. Thank you. The next question is coming from Bruce Galloway from Galloway Capital. Bruce, your line is live.
Hey, Jack. Congratulations. It seems like you're getting a lot of traction. Just to quantify the size of these contracts and some of the new additions, you know, you know, the $23 million hyperscaler that you announced in April, I guess that was the one that was doing $23 million a year that extended it for three years. So, that alone is a $43.5 million add-on from the $23 million, which on a base of $86 million last year, that's over 50% growth rate just from one customer. Can you give us some quantification on some of these other contracts, how big they could be and how much they can scale to?
Sure. So, you know, as I mentioned, we're now working with seven big tech companies. And we believe when we look across that portfolio that they are all spending significantly, as significantly as the company that you referred to, on these technologies. And, you know, we believe we have an opportunity to scale with all of them. Now, will all of them become as big as this one? That would be wonderful if it were to happen. I don't know that it will. But we believe we're certainly very well positioned. We brought in another two customers this quarter, which are very significant players and appear to be very eager to work with us. And in terms of, you know, when you start adding up the numbers and adding up what's possible, we believe that the 40% growth target, which doubles what we provided last quarter, is still a reasonably conservative target. We have lots of opportunity in flight. We're taking conservative view of our pipeline in order to support our growth guidance. And it's a very exciting time in our business right now.
So looking at your current infrastructure, your personnel and your installed base, I mean, do you have the capability? I mean, adding three million in personnel and three million in CapEx seems a very modest amount for the scalability and the upside that you have with all these huge contracts coming through.
So the investments that I spoke about were investments in the recruiting costs associated with adding the headcount that is required for the near-term contracts to get to that 40% growth. And, you know, other investments that we're making in sales, marketing, and product development, I'm not including in those numbers the costs that we would be incurring as cost of goods. But again, from a cost of goods perspective, I think you should look at it as an opportunity to drive, you know, in the services area, probably about 37% adjusted gross margin. And that would soon be the costs of producing the revenue.
Okay. And also, as far as the cash generation, you generated like $5.2 million in cash. Is that mostly from receivables or is it pure cash from operations or a combination thereof?
Yeah, I mean, it's cash from operations that include receivables. So as we build, you know, we then collect.
Okay, great. Thanks. Excellent job. Thanks.
Thank you. Thank you. And that does conclude today's Q&A session. I will now hand the call back to Jack Abahoff for closing remarks.
Thank you. So, yeah, we're off to an exciting start for 2024. And my team and I are tremendously energized by what we believe we will accomplish in 2024. We grew revenue by 41% year over year in Q1. We anticipate a substantial sequential revenue increase next quarter. And for 2024 overall, we have raised our guidance from 20% to at least 40% year over year expected organic-only revenue growth. Today, we announced yet another substantial customer win, this one worth approximately $23.5 million of annualized revenue. This is on top of the win with the same customer we announced a couple of weeks ago, valued at approximately $20 million of annualized revenue. We also announced the signing of two additional Big Tech customers, both of which we believe will contribute to 2024 revenue growth. Longer term, in addition to expected or continued expected growth from our Big Tech customer base, we expect increased market demand from enterprise customers that we hope will continue to accelerate our growth trajectory. We're super excited about the work that's underway. We are laser focused on creating long-term shareholder value. And thank you all for attending today's call. We'll be looking forward to our next call.
Thank you. This does conclude today's conference. You may disconnect your lines at this time. Thank you for your participation.