This conference call transcript was computer generated and almost certianly contains errors. This transcript is provided for information purposes only.EarningsCall, LLC makes no representation about the accuracy of the aforementioned transcript, and you are cautioned not to place undue reliance on the information provided by the transcript.
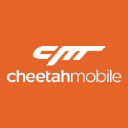
Cheetah Mobile Inc.
6/7/2024
Good day and welcome to the Cheetah Mobile first quarter 2024 earnings conference call. All participants will be in a listen-only mode. Should you need assistance, please signal a conference specialist by pressing the star key followed by zero. After today's presentation, there will be an opportunity to ask questions. To ask a question, you may press star then one on a touch-tone phone. To withdraw your question, please press star, then two. Please note, this event is being recorded. I would now like to turn the conference over to Helen, Investor Relations for Cheetah Mobile.
Thank you, operator. Welcome to Cheetah Mobile's fourth quarter 2024 earnings conference call. With us today, our company's chairman and CEO, Mr. Fu Shun, and director and CFO, Mr. Tom Munchen. Following management's prepared remarks, we will conduct the Q&A section. Before we begin, I refer you to the safe harbor statement in our Urgent Treaties, which also applies to our conference call today, as we will make forward-looking statements. At this time, I would now like to turn the conference call over to Chairman and CEO, Mr. Fushun. Please go ahead, Fushun.
Hello, everyone. Thank you for joining us today. This is our first earning course since November 2021. And we are excited to share our progress as we resume our quality updates. Cheta Mobile is making changes. We are moving from focus on 2C to 2B. In Q1, our revenue from AI and others or enterprise focused increased by 62% compared to last year, and 36% from the previous quarter. Now these revenues make up 43% of our total revenue. We expect this to grow to about 50% by the end of the year, making significant steps in our transformation. Our recent acquisition of Beijing Orange Star, an AI service provider, was an important move. It gave us a skilled sales team, strong ties with business customers, and end-to-end capabilities for LMS, including model training, file training, developing LMS-based apps, and enhancing service robots. a new touch for interacting with end users and customers in the AI era. With OrinStar, we are now focusing on making customer enterprise apps with LMS and introducing LMS-powered robots for specific business needs. with the two main reasons for this focus. First, market opportunity. Unlike competitive 2C market, enterprises are increasingly choosing LRM-based apps on private cloud due to data security concerns. However, they face challenges in developing paired apps and presenting substantial opportunities in China's enterprise sector. Second, signatures. Bringing together Cheetah and Orange Star allow us to combine our app enterprise with AI skills. After capturing the market opportunities by sharing By selling robots to business, we can even find new ways to use LLMs to improve efficiency. We are using product-driven approach to enhance our LLM compatibilities. This is why we focus on the 10 parameters, LLM segments, and avoid large upfront investment in GPUs. We believe that a trillion parameters LM is unnecessary. And the enterprise can deploy and use 10B LMs on private clouds at lower cost. Over the past few months, we changed 14B parameters foundation models from scratch, which has been approved by authorities for a large-scale rollout and ranks among the top of various lists. Additionally, we are fighting nearly all the open-source foundation models to offer more options for our customers. all without significantly increasing costs. Furthermore, we have seen positive developments by integrating LRM-based apps into our service robot. In particular, our delivery robot can now interact better with users, leading to increased demands. especially in Japan and South Korea. Currently, our overseas revenue has surpassed domestic revenues and continues to grow steadily. With LMS, we believe the features of our service robot will expand even further. I would also like to highlight how we assist our customers in using LRM-based apps efficiently. For example, we helped Hun Dun University develop LRM-based QA feature for its app, improving user experience. We also developed LRM-powered customer service features for another customer products, including WeChat mini programs, apps, and our service robot. This service is now available in Ningxia, helping local residents apply for housing funds. We are also working with enterprise in China's Branching the industry to improve management professionally with LMS-based apps. In the early stage of LMS-based app development, we closely work with our customers to understand their needs. Identify areas for improvement with LMS. Find the most appropriate LMS. Find it. and develop customer apps. This process helps us standardize some LRM-based apps and capabilities, particularly in customer service, enterprise management, and training, which we can replicate to more customers. As a result, we are monitoring monitoring customer feedback and certification. Additionally, all the applications can be incorporated into our service robot. Our long-term business model in LMS year, we were involved in selling robots and offering value-added service. As we focus on building LRM-based apps for enterprise, we will shift our resource from our agency internet business to AI business. This will improve the operating margin of our internet business, which we use as a financial performance metric. In summary, LRM is a once-in-a-generation opportunity. With Orange Star and our clear strategy, we are now confident in our direction. We would like to emphasize that we don't want to set short-time revenue growth targets, but we are aggressively prioritizing our customer satisfaction and building light-house projects By doing so, we believe we will establish a new growth engine to drive sustainable long-term growth in both revenue and margins over time All we need is a bit of patience We thank you all dedicated employees for their hard work in making this happen. Thank you.
And Thomas. Thank you, Fuzhou. Hello everyone on the call. Please note that unless stated otherwise, all money amounts are in RMB terms. Today, I am going to talk about two topics. First, our continued investment in large language models. or RLMs, resulting in abundant operating loss for the quarter, while total revenue has resumed its increase. Second, our healthy balance sheet. First, we are investing in RLMs. We aim to help enterprises quickly develop RLM-based new apps. As Fuzong mentioned in his speech, Our acquisition of Orange Star has allowed service robots to become a key revenue contributor to the segment of AI and others. In Q1 of 2024, revenues from AI and others increased by 62% year-over-year and 36% quarter-over-quarter to 81 million, accounting for 43% of total revenue in the same period. Driven by contributions from Beijing Orange Star, our total revenue increased by 12% year-over-year and 14% quarter-over-quarter to $190 million. This acquisition also allowed the two teams from Cheetah and Orange Star to work more efficiently together to better capture the opportunity in RLMs. as we help Chinese enterprises develop apps on RLMs to boost productivity. We expect this will lead to a substantial growth in revenue over time. In addition, RLMs are enabling us to improve the product experience provided by our service robots, which are now more capable of answering users' different inquiries. This enhancement has strengthened our competitiveness and should drive the sale of our service robots over time. In Q1 of 2024, our total non-GAAP cost and expenses increased 21% year over year and 19% quarter over quarter. And non-GAAP operating loss was 66 million in the quarter. up from 42 million in the same period last year and 49 million in the previous quarter. This is primarily due to the investments in RM and mentioned earlier. Through Beijing Orange Star, we acquired many R&D talents and 2D sales personnel, which are very important for us to capitalize on the opportunity in this sector. As of March 31st, 2024, we had about 860 employees, up from about 720 a year ago. We are also renting GPUs for model training and fun tuning. Excluding the impact of the aforementioned investment in our amps, our cost and expenses as well as our margins remain stable. For example, excluding SPC, our operating profit for the internet business was 7.9% in the quarter, up from 3.1% in the same quarter last year. As we continue to review our product portfolio and remove products that did not address user pinpoints, we will continue this approach moving forward. At the same time, we will continue to invest in talent, both in R&D, specialized in LRMs, and to be sales personnel, to help us seize the LRM opportunity to build a new growth engine for Cheetah. Our investments will be backed by our strong cash reserves. At the same time, we will continue to increase our operating profit for the internet business. Secondly, Cheetah Mobile has a healthy balance sheet. As of March 34th, 2034, we had cash and cash equivalents and short-term investments of about US dollar 250 million. In addition, we had about US dollar 130 million of long-term investments, which include several holdings in well-known entities, such as metasol.cn. Lastly, Inland is the practice of comparable China-based companies listed in the U.S. capital market. We have decided not to provide revenue guidance going forward. Thank you.
Everyone, for today's call, management will answer questions in Chinese, and an AI agent will translate management's comments into English in a separate line. Please note the translation is for convenience only. In the case of any difficulties, management's statement in Chinese works well. If you are unable to hear the English translation, A transcript in English will be available at our website within seven working days. Thank you so much. Operator, please now take questions. Thank you.
We will now begin the question and answer session. To ask a question, you may press star then one on your touchtone phone. If you are using a speakerphone, please pick up your handset before pressing the keys. If at any time your question has been addressed and you would like to withdraw your question, please press star then two. At this time, we will pause momentarily to assemble our roster. The first question today comes from Nancy Lu with JP Morgan. Please go ahead.
Um,
Let me answer that. I think our goal is to completely implement the transformation of our strategy this time. In other words, after a few years, R&D has become a core business of 2C, gradually turning to 2B as the core business and capability of the company. And the main catcher of 2B is the technology wave of artificial intelligence and large model this time. What we really want to do is to make the application of artificial intelligence better. This is one direction. This direction is the core strategy of our entire company. And then we have a new company called Slogan, which is that we want to become a new productivity provider in the era of artificial intelligence. Of course, this tool is a new tool, a productivity tool provider. This productivity tool is mainly this time we are mainly in the 2B industry. Here, you asked about these few points. We think it's still a product. Although AI is very popular today, there are not many products that can really land on the ground. Maybe the technology of the big model Thank you.
Operator, please move to the second question. Thank you.
The next question comes from Thomas Chong with Jefferies. Please go ahead.
晚上好,Maple移動是一家土C業務的核心企業。 現在企業要轉型土B,做檔案模型是有花的部署。 做機器人的副總理的信心來自於哪裡? Thank you for your question. I think confidence comes from practice.
Last year, at the end of the year, we acquired LWC. At first, LWC was also an investment company. I also spent a lot of effort to assist LWC in this. Because LWC is a trading market. So, during this process, they have a team, and we also participated. During the acquisition process of LWC, we also learned a lot of experience. And then I myself, you said 2B is different from 2C, we also spent a long time to learn, including a lot of effort to maintain customer and customer relationship. I think the most important thing is to create an organization capability suitable for 2B. We have also spent a lot of time in various ways in the past six months. Another thing I want to say is that in addition to the acquisition of LWC, we actually have A few years ago, the whole of Alibaba also did a lot of work on Tuobi, including a business called JuYun in the United States. The business is to provide cloud services to various companies, including Amazon and Google. We are also Amazon and Google's top partner in China. So at that time, we had already begun to continuously explore how to communicate with Tuobi's customers, and how our organization could adapt to the market of Tuobi. It should be said that it is true that 2C to 2B is very difficult to transform, but our management team, including myself, has spent a lot of energy not only to learn but also to practice. You said that it takes time to maintain the customer relationship with the client. Of course, because our organization has already built something similar to Huawei's Iron Triangle, we have a special position like ARFR to serve our customers. I spend time to maintain not the customer relationship, but more communication with customers to get their needs. Because only by understanding the customer's needs can we make the client's business better. This is what we have learned in the past few years. As for the layout, I think our idea today is that first of all, we have to be good with the standard customers. We now have a few industries that are dealing with top-level customers. This delivery is very important to us. Although we are doing 2B, but the 2C user experience is still our destiny. It will definitely make our customers feel that this is enough. We can provide him with enough services and products to satisfy him. After meeting the target customers, as I mentioned earlier, we can provide some standards This is equivalent to standardization, and it can be copied. Secondly, because of the group construction of 2B, our customer relationship can be used on the application of the large model, and also on the application of the robot. There have been many cross-examination cases here, which are purchased jointly by the large model and the robot customer. So I think that now we Operator, please move to the next question. Thank you.
The next question comes from Betty Wei with Citi. Please go ahead.
Thomas will answer. Vicky, thank you for your question. Most of these courses are related to our advertising business. Our ad agency business is to help many Chinese advertisers buy ads from several major overseas online advertising platforms. Since our income is only the fee for advertising, the total amount of the customer's purchase and payment to the advertising platform is recorded separately into the Pre-Payment and Payable items you mentioned earlier. This business is actually a currency business, and we have been operating for nearly 10 years. In these 10 years, we have formed a very strict mechanism to evaluate the performance of advertisers and to manage our income and wealth accounts. We are very careful about the cash management of this business. Thank you.
Operator, please move to the next question.
The next question comes from Miranda Zhuang with Bank of America. Please go ahead. Miranda, your line is open. You may ask your question. It appears we are unable to connect with Miranda at this time. So the next question comes from Karen Kong with TS Securities. Please go ahead.
Good evening, Mr. Wang. Thank you very much for having such an opportunity to ask a question today. I would like to ask Vice President, including Thomas, because in fact, the market is more concerned about prey, which is also the most growing and future space for development in our prey. And then, as Vice President just mentioned, we prey on this large model business and machine business. I would like to ask this question. Our market is also more concerned about our customers. Then one is from the shareholder's, our shareholder's shareholder's shareholder's shareholder's shareholder's shareholder's shareholder's shareholder's shareholder's shareholder's shareholder's shareholder's shareholder's shareholder's
Thomas, please answer this question. Thank you for your question. When it comes to listed companies, after the acquisition, the main focus of the listed companies' business is the main focus of the listed companies' business. But as a listed company, we always strive to create the greatest value for the shareholder. Regarding the listed companies' planning, we will fully assess the opportunities of various capital operations. including the possibility of letting subsidiaries go public on their own or carry out independent financing. Our goal is to improve the performance and market value of our clients in an effective way, and thus further improve the share price of the entire company. In each of the role models, we will consider the long-term interests of market environment, company strategy, and the long-term interests of our clients, ensuring that each of our operations can bring the biggest return to the shareholders. Thank you.
Okay, operator, please move to the next question.
The next question comes from Miranda Zhuang with Bank of America. Please go ahead.
to make some project-based products, and the results are not very good. And I also saw that the market is shrinking recently. So I really want to know what innovations the company has made in the deployment of the large model. What is the income and profit rate of the project made by the company? And then you can help us to better understand that in this era of large models, Why do we think that corporate applications need to be customized to deploy? After all, we have seen more and more manufacturers in the past few days provide more standardized models, these adjustment tools, and then also do all kinds of low-cost actions, and even do it for free. In such a way, Okay, there are a lot of questions. Let me answer them briefly. The first one is about cloud companies.
To be honest, I don't know much about it, because according to what I know about some cloud manufacturers, they make a huge amount of cloud privatization, which is actually different from the privatization we talked about today. But in fact, cloud manufacturers have been doing this for a long time to provide enough good deployment services to their customers. It's just that, for example, the deployment of large companies like Amazon, their staff is already at a very high level, so they let their partners complete it. We have a lot of projects like this. And because of our partners, the cost is lower, so we have a lot of profits. Today, we talked about the income and profit rate of the project. Today, we will talk about how we can really help the business. I just mentioned that we are still working on the benchmark. We don't think much about the profit rate right now. And then the next question is why do large-scale enterprises need to be self-sufficient? Because the bigger the enterprise, the more it will consider data security. And today, you use public-owned large-scale, you really pass on to him a lot of internal articles of the enterprise, especially some sensitive articles. In fact, most enterprises are are very concerned about this. Because the internal ability of the big model comes from the data. After the data of the Internet is caught, the data inside the company is also a very important data source. So what we see is that at least the customer level is very concerned. And basically, companies that are on a certain scale are all asking for the personalization deployment of the big model. What is the difference between us and the previous cloud? We are doing personalization. First of all, the cost of personalization deployment of the big model itself is not high. It is not a complex deployment system. In fact, today's big models, basically you use some servers, not two servers, this big model can be freely deployed. So the cost of deployment itself is very low. The second is that when doing project management, because today we are doing this AI landing, we are not included in the big model. The big model has a different ability from the previous applications. Its own reasoning and understanding is relatively strong. So this allows us to contact other industry customers. Compared to the previous era, including the previous era, not only Yunla, but also SaaS. In fact, our ability to cross the field is much stronger than before. In other words, if I didn't know enough about the industry, it would be difficult for me to deploy. But because of the model, I can understand it myself. I don't know if you understand this or not. It will understand a lot of professional knowledge by itself. So when you look at the workload of our deployment, it's not called deployment, it's called helping it to do the application. The workload will be much less than before. And once it forms this kind of center stage, its profitability will also be much stronger. For example, we talked about doing public funds for a political project. People can do public funds and they can ask questions directly. We started to do it for a long time. But when the second client comes, our deployment may also take two or three weeks to complete. The third one is what you asked. The large model of cloud manufacturers is constantly reducing costs, even for free. Now most of the free models are open source. When the open source model is really being used, most of the customers we meet are asking for their own privacy deployment. When privacy deployment is divided into two aspects, one aspect is called internal privacy deployment, which is high in terms of security. There is also a kind of cloud-based privacy deployment, which is that I set up a model on the cloud, but the model is only for me to use, so it cannot be used by others, and the data cannot be crossed. This kind of deployment is also called privacy deployment. So you just asked about that. In fact, it is mainly a data security consideration. Of course, in fact, if you have a lot of large-scale applications, the cost of deploying a machine is lower than that of constantly showing tokens. In fact, there will still be some advantages. The third is that what kind of enterprise and that fit? We think it's this. Please move to the next question, thank you.
The next question comes from Zhai Lulu with Voitai. Please go ahead. .
Because the robot we make is not an industrial robot, it is an automated robot. We make the robot for the service industry. Our customer group is the corporate users. And after we launched the social hunter, they are more of an agent system. Their agents not only do the robot business, but also do a lot of this corporate information implementation, information deployment business. So only from the channel point of view, a large part of it can be used. Secondly, from a technical point of view, this big model is the brain of the robot. In the past, the robot industry, in addition to industrial robots, other robots have not developed well enough. A very important point is that the robot's brain capacity has not risen. Now, why is GSM so popular? Actually, it's because of the improvement of decision-making and judgmental power brought by the large-scale model. Now, everyone thinks that the service robot industry, whether it's called a humanoid robot or a humanoid robot, everyone thinks that its future will be very good because of the breakthrough of the large model. So what is our relationship? On the one hand, we just talked about the relationship between the customer and the client, which can be reused. Most of them can be reused. Once you establish a connection with a 2B business client, they will be interested in your big model. When they talk to your big model, they will feel that your robot can also help them in many jobs. The second and more important thing is that if we don't build our big model well, our robot will lose its competitiveness for a long time. Because our robot is not a hardware industry, but a real decision-making industry. Now we have a better understanding of how to use the ability of the large-scale model on the robot through the training of the large-scale model, including micro-tips, applications, and so on. We have now started to do some training in the field of giant-scale intelligence, which is no longer simply doing large-scale model training. In other words, the ability of our robot to combine giant-scale intelligence and large-scale model will be continuously extended. What we can see in the current stage is that we have been doing this for many years, which is called voice interaction. That is, we have been doing this for many years, which is called voice interaction. We have been doing this for many years, which is called voice interaction. We have been doing this for many years, which is called voice interaction. We have been doing this for many years, which is called voice interaction. We have been doing this for many years, which is called voice interaction. Then, And this accurate improvement is not due to the previous large number of artificial ones to do the Q&A team or something, but now as long as you give him some documents, the robot's understanding of this is able to do it to a very high level. So at this stage, it is obvious to see that the demand of the robot user is increasing in the context of this introduction. Especially the large-scale model itself is multi-language. In the past, we used to have only D-Song in overseas robots, because the work of a cross-language language is very big for us. But now there is a large-language model, which is naturally multi-language. So we will also launch this kind of robot with language interaction overseas in the next step. Of course, in the long run, we are also doing some machine currency training, so that the robot can do some work. But this still needs some time. Thank you.
Next question, please, operator.
The next question comes from Boris Van with Fernstein. Please go ahead.
Hello, Mr. Van. Thank you for giving me this opportunity to ask a question. I have two questions. One question is about the scene of our down-to-earth deployment. The competitiveness is actually quite high. And many companies with a lot of resources The second question is about AI talents. I'm curious, I want to ask you about AI talents. What do you think about those trends? Thank you.
This is a good question. I would like to emphasize that we started in 2016. At that time, artificial intelligence was already being developed. In 2017, Alibaba called out the slogan of all-in-AI. We also cooperated a lot with Alibaba. So it should be said that our experience did not start from last year. Although the original model and the previous model have some characteristics that are different, but its underlying neural network, this transformer, we have already used it on the earliest TTS, on the sound model, there is a part of the transformer that has been used. So our entire team has a long-term understanding of this transformer. I think the advantage of micro-chip is that it can be done in detail. Because micro-chip itself requires more than a hundred thousand words to be prepared. Also, it requires a lot of thought and detailed management in terms of data refinement based on the scene. It also requires communication with users. Here I think if we talk about competitive advantage or who has what competitive advantage, I think in such a fierce market, it is difficult for a company to say that it has any unique and unpleasant advantages in terms of technology. I think our more advantage comes from the combination of customers and the market, which is what we really focus on. This is a fast-paced process. It's not that you can't do it at a certain point. So we constantly emphasize the user's reputation and the importance of landing projects. Did someone ask if the model can be updated? Yes. I'm sorry. To be honest, the capacity of the large-scale model today has a certain push, but it is different from the need for the scene of the enterprise. uh uh Is it the model that solves it, or is it the application that solves it? We found that even if it is a model like ChatGPT OpenAzon, you can only satisfy the customer with a professional knowledge question in many companies. This is our own experience. So, we need to customize some components according to the customer's needs. We need to work on the same model. After working on the same model, the user can really achieve the role of the so-called digital employee. In fact, it seems that the market lacks a real solution that can satisfy customers. This is our understanding of the market. So you asked about the competitive advantage. In fact, we are going to explore the needs of customers in depth, and then make the details better. As for the relevant talents, first of all, our leaders, because it involves We don't talk about it, but we have published a paper on it. It has enough knowledge and the potential of this industry. Then, in terms of the specific implementation personnel and some algorithm engineers, in fact, there are quite a lot of talents in China. In this regard, it is not too difficult to recruit such people in the market. And we are not prepared to compete in the big model with such a large number of parameters and extreme capabilities. The next question comes from Richie Sun with
HSBC, please go ahead.
Then how do we solve this kind of continuous delivery? Then how do we look at the cost of this kind of delivery for some of the products we provide? Or how can we maintain the efficiency of this delivery in terms of our products? Also, when we chose a large model based on the business scenario for our customers, we used some micro-tooling and application. But in fact, the model is very fast. When these models are folded, will it completely cover our ability to micro-tool large models? Thank you. Yes.
Thank you very much for your question. There are a few concepts that I would like to talk about first, such as micro-tuning and application. These two are different concepts. In fact, in the context of most enterprises, it is not necessary to do a large-scale micro-tuning for the enterprise alone, because the basic capabilities of the current model It's called the way to write an application package to combine the needs of the model and the enterprise. When the model is copied, the application package is not outdated. Because the application package is more about combining some systems within the enterprise. For example, if you ask a big model a question, for example, what kind of certificate should I get today? It will say what kind of certificate you want to provide, right? You tell me your ID number. Then after you tell him, he will check the ID number. This is part of the application. After writing the interface of this application, as long as your model is relayed, it has no impact on it. This is the first one. The second one is that in fact, after the ability of the model is enhanced, the smoothness of the application will be increased in accuracy and various aspects of user experience. I don't think it's a conflict at all. But now it looks like the ability of the model is increasing again. It is also impossible to know the needs of each enterprise. It's different when you look at the needs of all kinds of enterprises. Today's models are all based on the data training of the Internet. For example, it doesn't know what the needs of the administration are. What are its internal documents? And what are the needs of the employees? These are all applications that need to be solved. Now it looks like this model has been upgraded. There are also a few open model upgrades recently. We are all very welcome. Because our applications can be done better on it. This is different from what we used to do. Some things are still different. The first one is the API interface. It won't work like this. The second one is that we still use the prompt to interact with it. After the model is upgraded, these things won't be affected. The model and the application are a relationship of cooperation. At present, in a very long time, Or I almost think that it is not possible to deploy a model online and the user can use it. In fact, there are still many opportunities for enterprise applications here, and there is also a great need. We can also look at companies like OpenAI, which has also opened GPTS and opened more API interfaces, allowing more enterprises to really combine their actual The next question comes from Wei Fang with Mizuho. Please go ahead.
Thank you for your question. I have a question about the chip. We know that the domestic high-end chips are still limited, right? First of all, I would like to ask you if you will continue to train your own big model. In addition, you are giving the clients, right? When these corporate clients help them to make the micro-chip, how do you solve the computing problem? Thank you.
First of all, we have a large model that we train ourselves. Our parameters are all in this. I just said that we are training a model with a scale of more than 100 billion parameters. We are still training a MOE model, but the parameters will not be much larger. Because we are more concerned about the implementation cost of the company. Because if such a parameter model is really used, it probably only needs a higher-end server to be able to use it. This is the first point. So we don't have that much demand for chips. Compared to the chip demand of the big model companies that do 1000 billion parameters or bigger parameters. Secondly, we think that today's big model training is indeed very short. We don't need to do repeated large-scale construction on it. In fact, we thought that the open source community would be very prosperous long ago. More and more open source models with good performance will emerge. Today, the situation seems to be the same. And this makes us choose models, not only our own models, but also many open source models that can be directly provided to customers. So we are not so worried about the chip problem. OK, thank you. Thank you.
Operator, we have no further questions. I'll end the call.
There are no further questions at this time. I'd now like to hand the call back over for closing remarks.
Thank you, operator, and thank you so much for joining our conference call.
Thank you. Thank you, everyone.
The conference is now concluded. Thank you for attending today's presentation. You may now disconnect.